Guidelines for Implementing Interpretable Machine Learning in Computational Biology
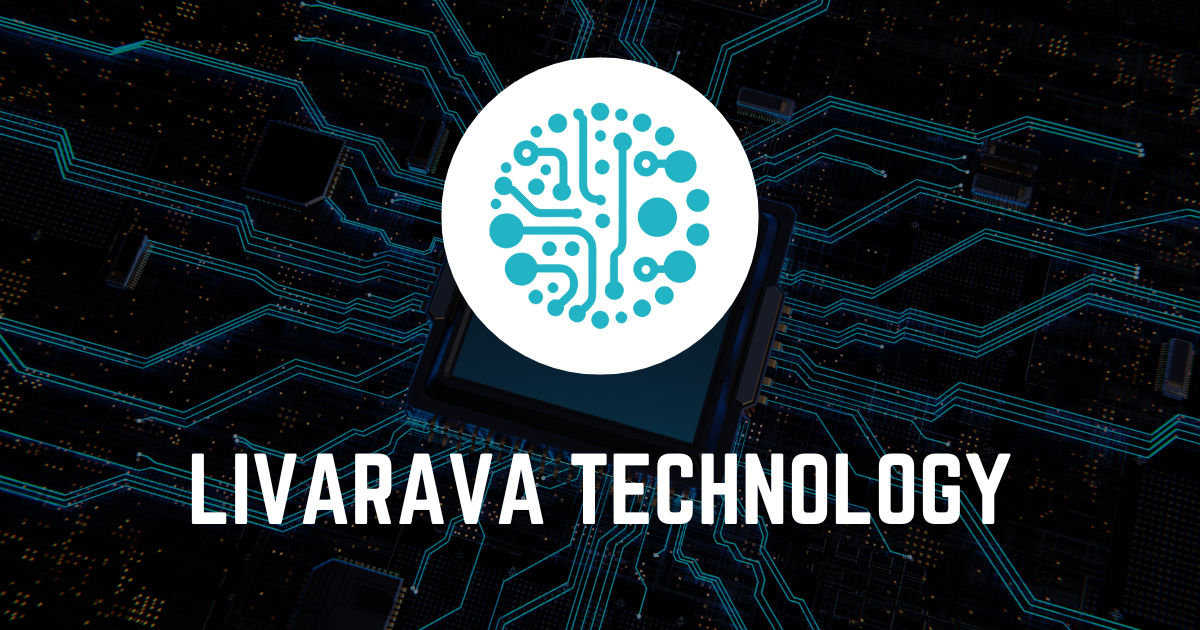
Introduction
Machine learning is a powerful tool in computational biology, enabling the analysis of a wide range of biomedical data such as genomic sequences and biological imaging. But when researchers use complex models, understanding their outputs can be challenging.
Importance of Interpretability
Interpretable machine learning methods provide insights into the underlying processes of biological systems, which is crucial for validating results and guiding subsequent research.
Guidelines for Use
- Utilize transparent models that offer clarity in decision-making.
- Ensure reproducibility of results to enhance trust in research findings.
- Leverage visualization tools for better understanding of model outputs.
Conclusion
By integrating these guidelines into their research, scientists can significantly enhance the application and understanding of machine learning in computational biology.
This article was prepared using information from open sources in accordance with the principles of Ethical Policy. The editorial team is not responsible for absolute accuracy, as it relies on data from the sources referenced.