Innovative Machine Learning Approach for Clustering Materials Based on Target Properties
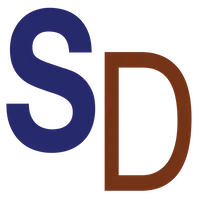
Introduction
Conventional clustering techniques often overlook crucial features of materials, leading to reduced effectiveness in analysis. This article discusses a novel machine learning method aimed at enhancing material property clustering.
Traditional Clustering Limitations
- Focus on basic features
- Neglect of target material properties
- Inadequate for advanced applications
New Methodology
This study introduces a machine learning approach that integrates more complex target properties. By prioritizing features such as band gaps and dielectric constants, researchers aim to ensure a more comprehensive and accurate analysis.
Conclusion
In summary, the implementation of this innovative machine learning technique could significantly transform how we understand and classify materials, prompting new discoveries and applications in the field of material science.
This article was prepared using information from open sources in accordance with the principles of Ethical Policy. The editorial team is not responsible for absolute accuracy, as it relies on data from the sources referenced.