Evaluating the Effectiveness of Reward Modeling in Generative AI Systems
Tuesday, 10 September 2024, 17:00
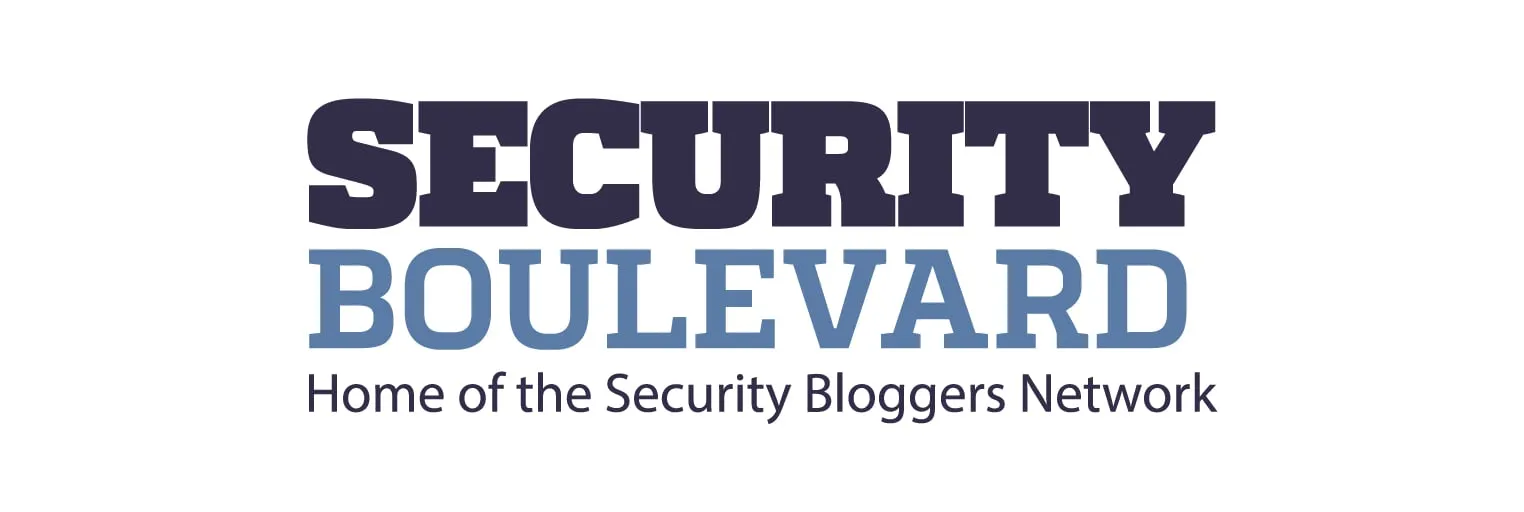
The Importance of Reward Modeling in AI
In the evolving field of generative AI, reward modeling serves as a cornerstone for optimizing machine learning algorithms. The latest research introduces a framework called SEAL: Systematic Error Analysis for Value Learning, crucial for understanding human feedback mechanisms.
Understanding Reward Modeling
- Reward modeling utilizes human insights to guide AI behaviors.
- This approach enhances performance and aligns AI systems with user values.
Key Findings from Recent Research
- Effective reward models significantly improve reward learning.
- Error analysis reveals common pitfalls in current modeling techniques.
- Implementing systematic methodologies leads to better alignment in AI responses.
For more insights and detailed findings, we recommend seeking out the complete research publication.
This article was prepared using information from open sources in accordance with the principles of Ethical Policy. The editorial team is not responsible for absolute accuracy, as it relies on data from the sources referenced.