Harnessing RAG for Trusted Generative AI Applications
Wednesday, 4 September 2024, 09:38
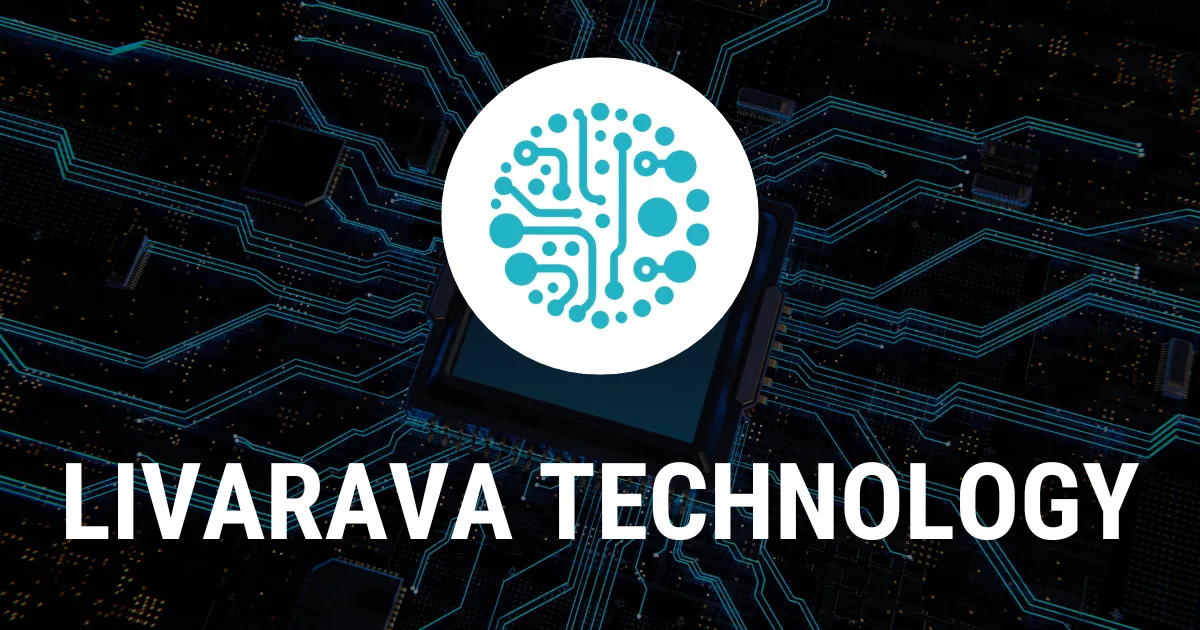
Understanding RAG in Generative AI
RAG, or Retrieval-Augmented Generation, is revolutionizing how developers create trusted generative AI applications. By integrating data retrieval processes into generative models, RAG enhances the quality and reliability of AI outputs.
Benefits of Using RAG
- Increased Trust: RAG improves user trust in generated content by using real-time data.
- Enhanced Reliability: Applying RAG ensures that generative AI outputs are grounded in accurate information.
- Streamlined Development: RAG simplifies the development process, allowing faster deployment of applications.
Key Considerations
- Designing a system that effectively incorporates RAG mechanisms.
- Training AI models on diverse datasets for improved output.
- Regularly updating data sources to maintain accuracy.
This article was prepared using information from open sources in accordance with the principles of Ethical Policy. The editorial team is not responsible for absolute accuracy, as it relies on data from the sources referenced.