Engineering Platforms for Scalable Machine Learning Data Architectures
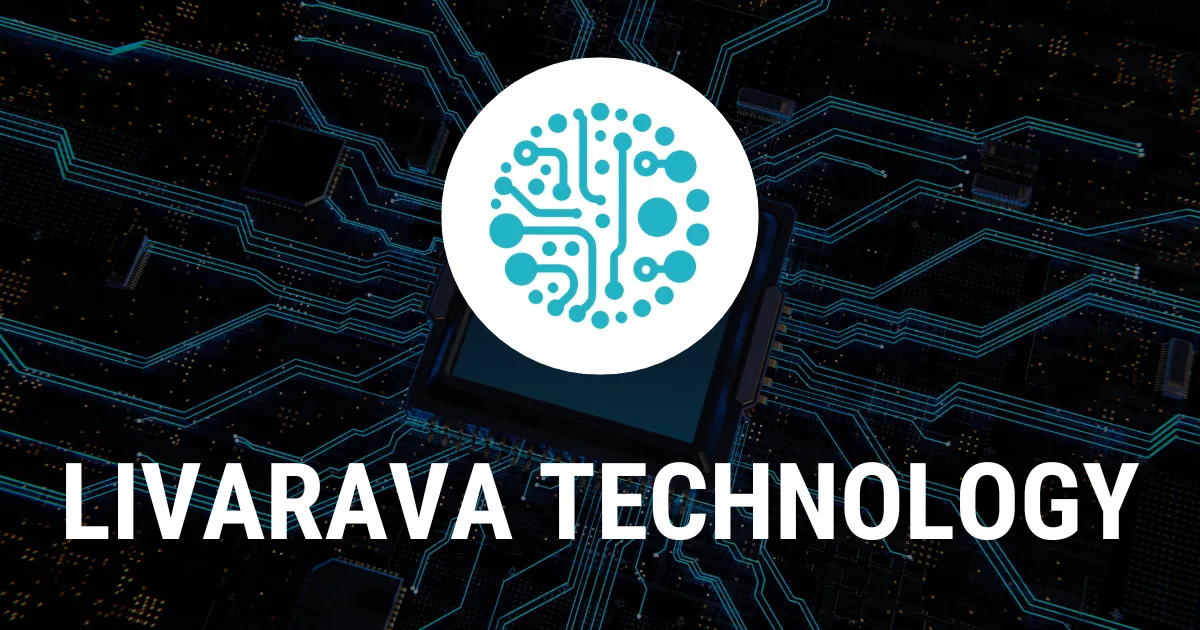
Engineering Scalable Platforms
Engineering scalable platforms is essential for innovating data architectures in machine learning. Due to the exponential growth of data, organizations are challenged to develop robust architectures that can handle this surge efficiently.
The Importance of Platforms in ML
Data architectures must evolve along with the technologies that handle them. The integration of AI-driven solutions is pivotal for delivering better performance and scalability.
- Flexibility: Platforms that adapt to changing data needs.
- Interoperability: Ensuring seamless integration with existing technologies.
- Efficiency: Minimizing resource consumption while maximizing output.
Future Trends in Data Architecture
- Cloud Solutions: The move towards cloud-based platforms is accelerating.
- Edge Computing: Enabling faster data processing closer to the source.
- AI Integration: Harnessing artificial intelligence to enhance architectures.
As we delve deeper into the requirements and advancements in engineering platforms, it’s clear that the future belongs to those who can innovate rapidly while maintaining efficiency in data management practices.
This article was prepared using information from open sources in accordance with the principles of Ethical Policy. The editorial team is not responsible for absolute accuracy, as it relies on data from the sources referenced.