How AI and Deep Learning Are Transforming Detection of Pulmonary Arterial Hypertension (PAH)
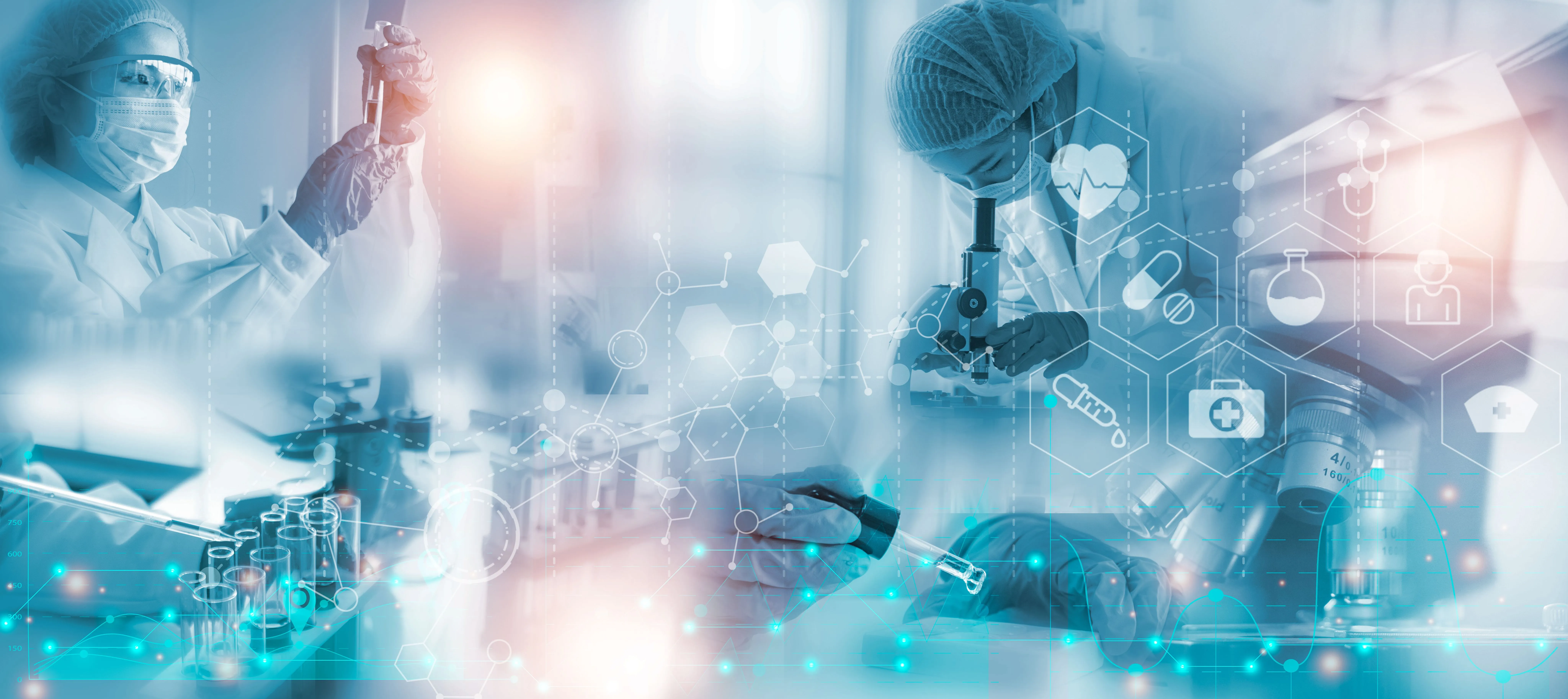
Challenges in Detecting Pulmonary Arterial Hypertension (PAH)
Pulmonary arterial hypertension (PAH) is a complex and life-threatening condition often diagnosed late due to traditional diagnostic methods being too slow and invasive. Right heart catheterization, the gold standard for diagnosis, presents significant barriers due to its invasiveness and cost. Recent advancements leverage technologies like AI and deep learning to address these challenges.
The Promise of AI in PAH Diagnosis
A pivotal study published in the European Heart Journal illustrates an innovative approach using 450 PAH patients to develop deep learning algorithms that could effectively assist in diagnosis. The accuracy achieved was an impressive 97.6%, demonstrating AI's potential in identifying this condition early. Dr. Gerhard-Paul Diller highlighted that this model could augment the capabilities of traditional echocardiography without fully replacing human expertise.
Combining ECG and Chest X-Ray Data
Another significant contribution came from research documented in the Journal of Imaging Informatics in Medicine. This study combined ECG and chest x-ray data from over 30,000 patients, achieving a negative predictive value of 98%, signifying its utility in clinical settings for initial screenings. Dr. Wen-Hui Fang emphasized that such high predictive power can lead to timely interventions.
Optimizing Patient Outcomes
- High Predictive Values: Advanced models using AI deliver substantial improvements in early detection.
- Screening Efficiency: AI can streamline the selection process for invasive testing, ultimately enhancing cardiovascular outcomes.
- Ongoing Research Required: Understanding AI's interpretability remains a crucial focus for future research, ensuring human experts can act upon AI insights.
In summary, AI and deep learning represent a significant step forward in addressing the diagnostic delays associated with PAH. Continued studies and integration of these technologies into clinical practice could lead to enhanced patient care and better health outcomes.
This article was prepared using information from open sources in accordance with the principles of Ethical Policy. The editorial team is not responsible for absolute accuracy, as it relies on data from the sources referenced.