Enhancing Cyclone Predictions with Machine Learning and Physics
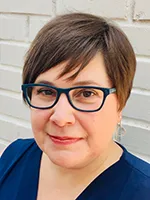
Revolutionary Techniques in Cyclone Prediction
The integration of physics and machine learning has given rise to a new era in cyclone predictions. Researchers have developed a hybrid modeling approach, combining traditional physics-based methods with sophisticated machine learning algorithms to enhance the accuracy of path and intensity forecasts.
The Hybrid Modeling Approach
This innovative method incorporates real-time data and advances in technical modeling, enabling meteorologists to predict cyclone behavior with unprecedented precision. The fusion of these methodologies not only improves existing forecasting models but also expands the potential for future research in atmospheric sciences.
Implications for Cyclone Preparedness
By applying these cutting-edge techniques, communities in cyclone-prone areas can bolster their disaster preparedness. Enhanced predictions allow for timely warnings, potentially saving lives and minimizing property damage.
Future Directions
- Improving data collection methods
- Further integrating machine learning with physics
- Developing international prediction models
As technology evolves, the intersection of machine learning and physics will continue to redefine how we approach natural disasters. To learn more about this significant advancement in cyclone modeling, stay tuned for further updates.
This article was prepared using information from open sources in accordance with the principles of Ethical Policy. The editorial team is not responsible for absolute accuracy, as it relies on data from the sources referenced.