Quantum Deep Learning Transformations in Rice Yield Forecasting
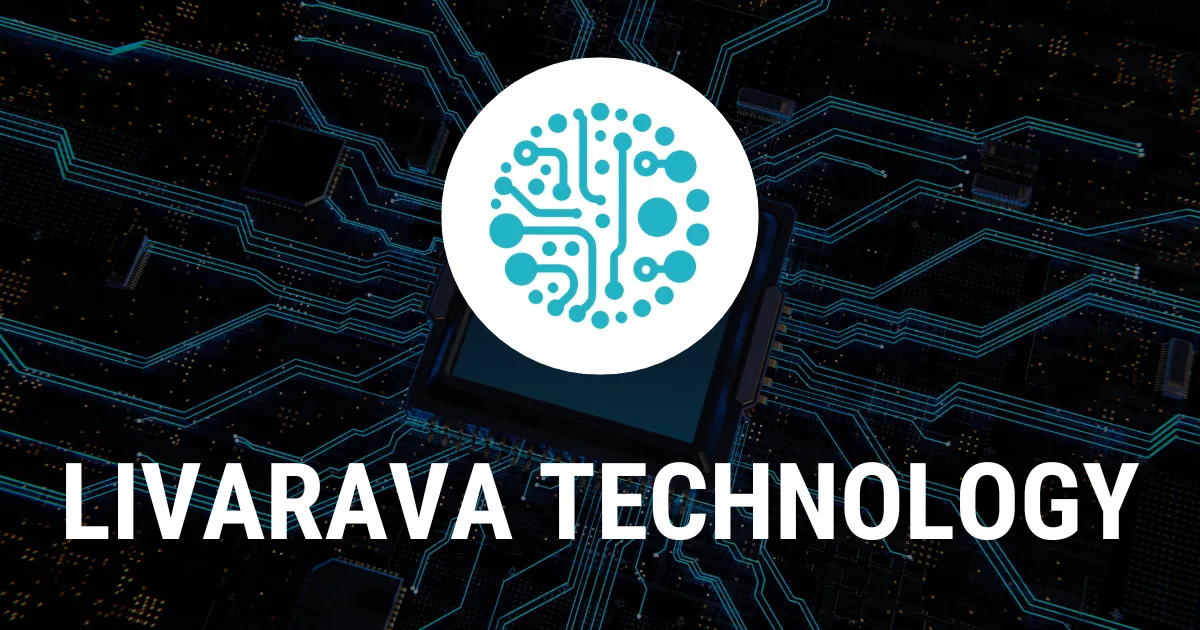
Quantum Deep Learning Innovations in Agriculture
In recent years, the blend of quantum computing and deep learning has emerged as a powerful force in various sectors. The latest breakthrough in rice yield forecasting showcases this fusion, presenting a hybrid model that incorporates quantum mechanics.
Hybrid Model Advantages
- BiLSTM Integration: This component enhances temporal prediction capabilities.
- XGBoost Techniques: These algorithms notably improve computational efficiency and prediction precision.
With the agriculture sector increasingly reliant on advanced technologies, utilizing quantum deep learning paves the way for more accurate forecasts, ultimately fostering food security.
Future Implications
The implications of this technology extend beyond rice farming. As quantum deep learning becomes more prevalent, we anticipate substantial shifts in agricultural productivity and operational methodologies.
This article was prepared using information from open sources in accordance with the principles of Ethical Policy. The editorial team is not responsible for absolute accuracy, as it relies on data from the sources referenced.