The Impact of Data Quality on AI Model Performance
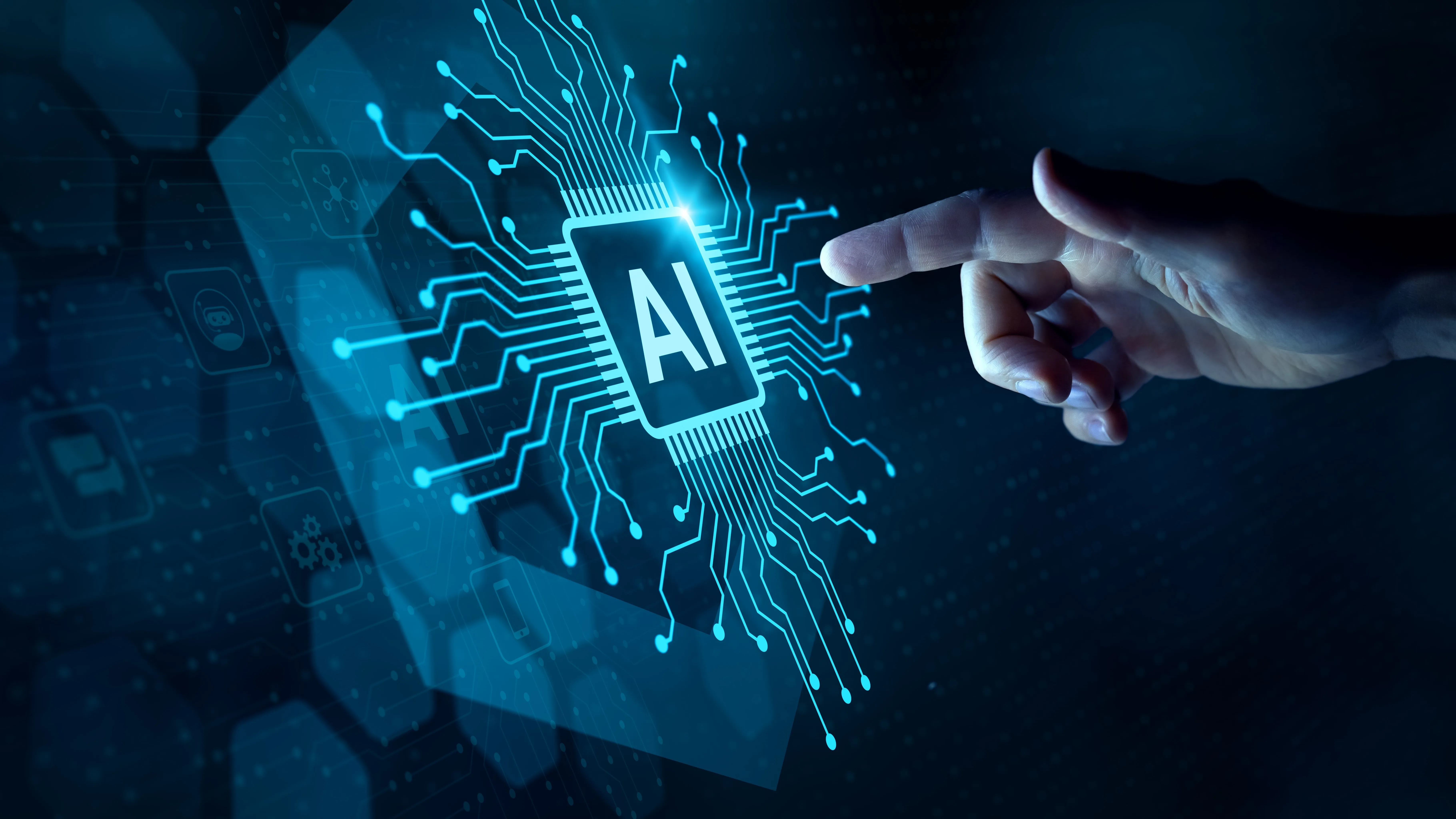
Understanding Data Quality in AI Models
The effectiveness of artificial intelligence models heavily relies on the quality of information that is fed into them. Poor data quality can result in models that produce biased and inaccurate outputs.
The Importance of High-Quality Data
To foster practical and successful AI implementations, it is crucial to focus on the following:
- Data Integrity: Ensure the data used is accurate and representative.
- Bias Mitigation: Identify and eliminate underlying biases in training datasets.
- Continuous Monitoring: Regularly assess and update data quality.
Conclusion
Organizations must make a concerted effort to enhance data quality to mitigate the risks of bias and hallucination in AI. By prioritizing data integrity, we can build more effective and equitable AI models that serve our society better.
This article was prepared using information from open sources in accordance with the principles of Ethical Policy. The editorial team is not responsible for absolute accuracy, as it relies on data from the sources referenced.