Identifying Vulnerabilities in Speech Emotion Recognition Systems
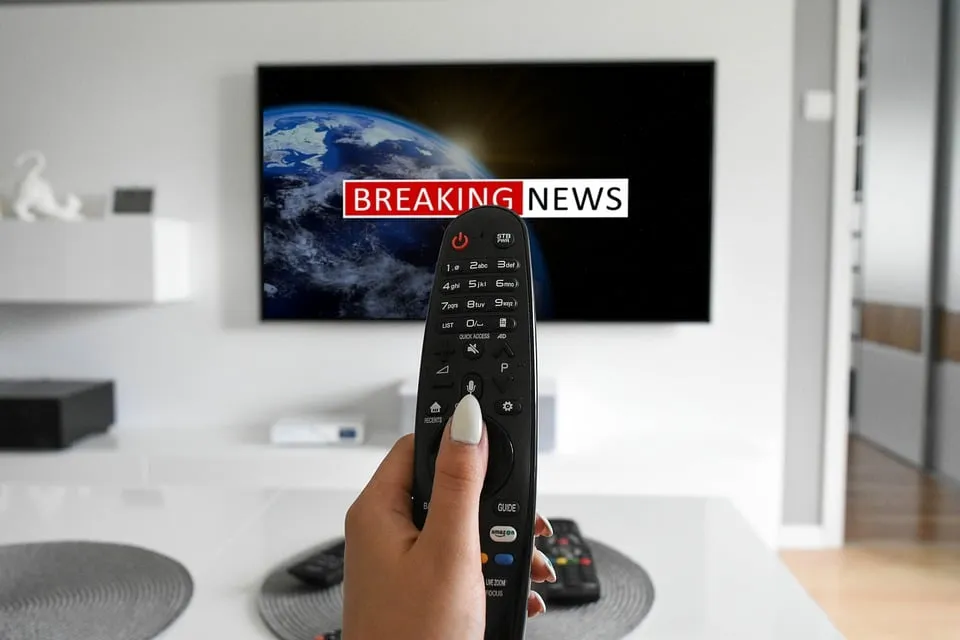
Understanding Speech Emotion Recognition
Speech Emotion Recognition (SER) utilizes deep learning techniques to interpret emotional cues from voice recordings. Although the technology is advancing rapidly, it faces significant challenges related to security vulnerabilities.
Current Vulnerabilities in SER Models
- Deep learning models have shown to be susceptible to attacks.
- Adversarial attacks can manipulate input data, leading to erroneous emotional interpretations.
Implications of Vulnerabilities
- Inconsistent emotional analysis could harm user trust in the technology.
- Potential misuse in sensitive areas such as mental health assessment or security screening.
Conclusion
To enhance the security of SER models, ongoing research and development are necessary. By addressing these vulnerabilities, developers can create more robust applications that maintain public trust and utility.
This article was prepared using information from open sources in accordance with the principles of Ethical Policy. The editorial team is not responsible for absolute accuracy, as it relies on data from the sources referenced.