Interpretable Machine Learning in Computational Biology: Insights and Strategies
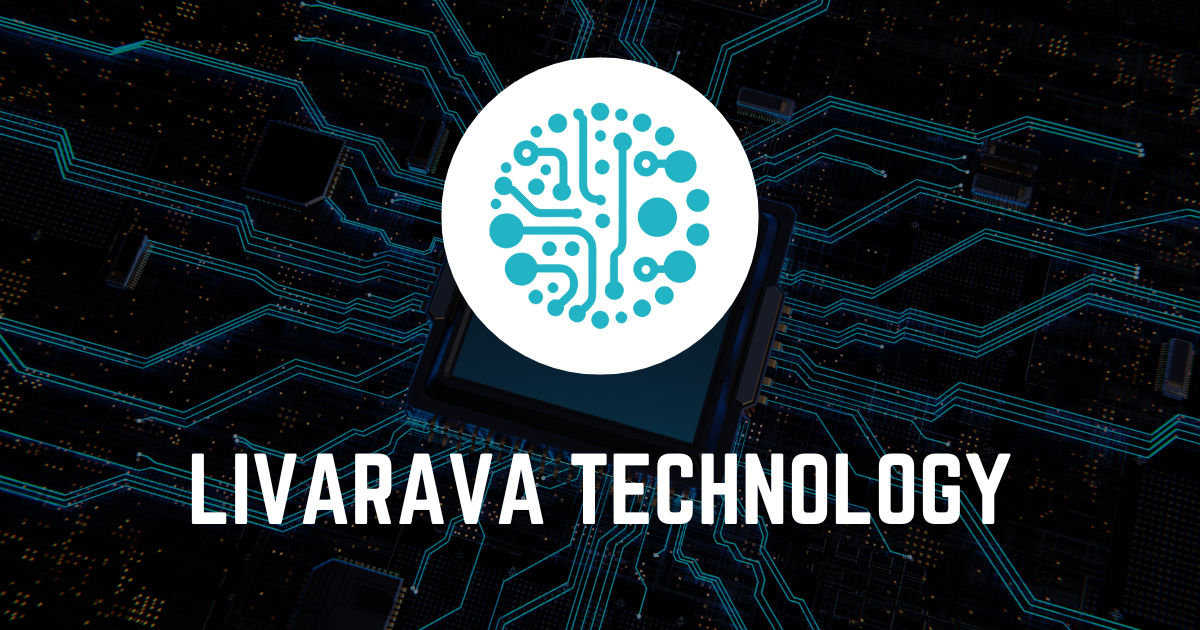
Applying Interpretable Machine Learning in Computational Biology
Interpretable machine learning provides a way to enhance the understanding of models used in computational biology. This article discusses the pitfalls and recommendations for utilizing these interpretability techniques effectively.
Recommendations and Opportunities
- Enhance Transparency: Focus on making machine learning models more understandable to researchers and practitioners.
- Use Established Frameworks: Leverage existing frameworks for interpretability to avoid common mistakes.
- Collaborate with Experts: Work alongside domain experts to ensure that the machine learning models align with biological realities.
Conclusion
Adapting interpretable machine learning in computational biology is essential as the field moves towards more sophisticated data analysis methods. By addressing pitfalls and following recommendations, researchers can pave the way for significant advancements in medical and biological research.
This article was prepared using information from open sources in accordance with the principles of Ethical Policy. The editorial team is not responsible for absolute accuracy, as it relies on data from the sources referenced.