Optimizing Feature Engineering for Better Machine Learning Outcomes
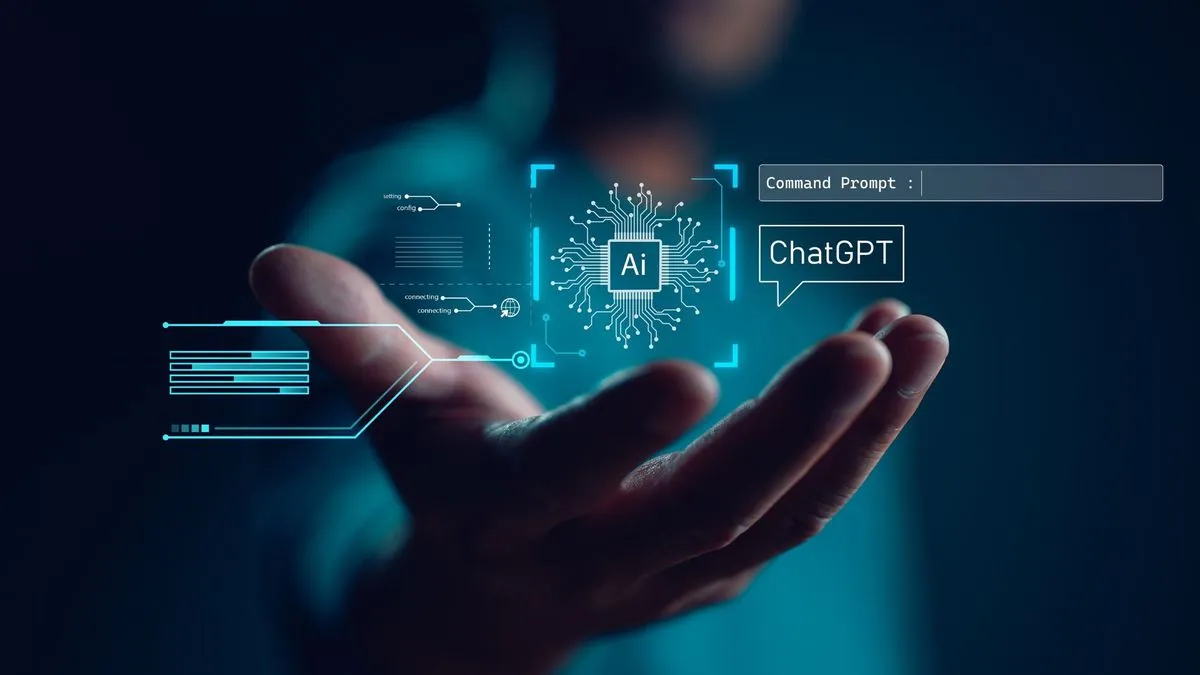
Understanding Feature Engineering
Feature engineering is a crucial step in machine learning that involves preparing and transforming raw data into a format suitable for model training. It is often considered one of the most complex pre-processing tasks in the data science workflow.
Simplifying the Process
- Utilize Automation Tools - Automating routine tasks can save time and reduce errors.
- Focus on Essential Features - Prioritize features that significantly impact model performance.
- Employ Domain Knowledge - Leverage insights from specific industries to guide feature selection.
Conclusion
By adopting these methods, developers can significantly ease the burden of feature engineering. This not only enhances efficiency but also contributes to building more robust and accurate machine learning models.
This article was prepared using information from open sources in accordance with the principles of Ethical Policy. The editorial team is not responsible for absolute accuracy, as it relies on data from the sources referenced.