Enhancing Text Embeddings in Small Language Models with Contrastive Fine-Tuning Techniques
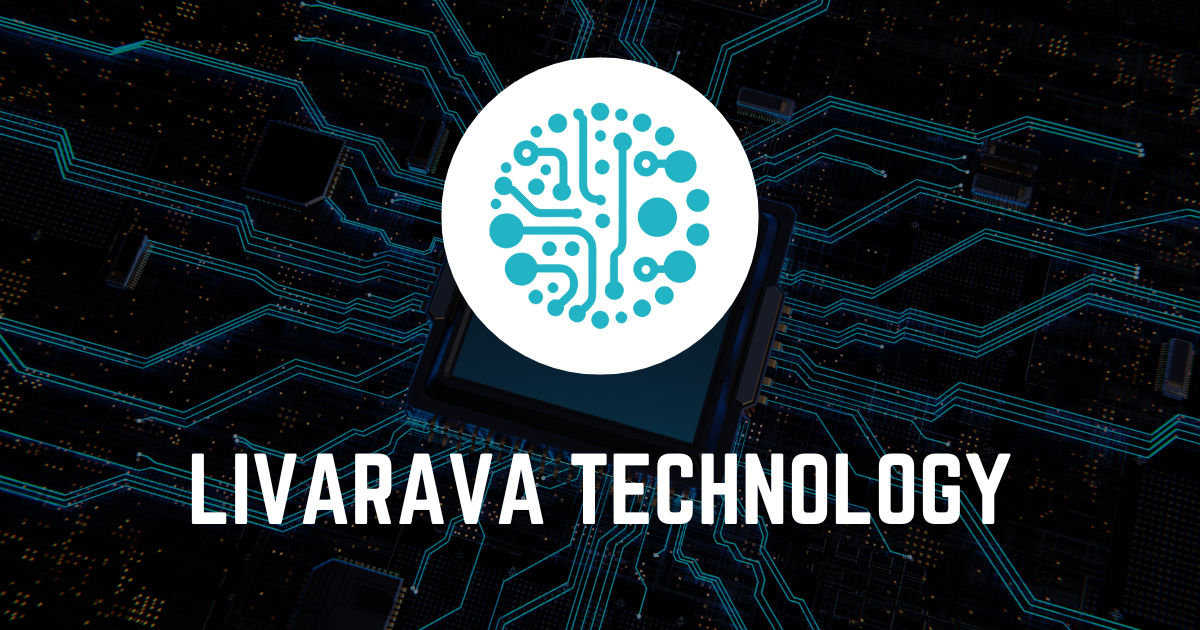
Enhancing Text Embeddings in Small Language Models
This article focuses on improving text embeddings within small language models using advanced techniques. With the rise of machine learning applications, the effectiveness of small models can be significantly boosted through innovative methods such as contrastive fine-tuning.
Contrastive Fine-Tuning Approach
- Comparison with traditional methods
- Significance of embedding optimization
- Implementation using MiniCPM framework
Conclusion
The findings suggest that adopting these enhanced techniques can lead to notable improvements in language model performance, making them more competitive against their larger counterparts. This paves the way for broader applications in real-world scenarios.
This article was prepared using information from open sources in accordance with the principles of Ethical Policy. The editorial team is not responsible for absolute accuracy, as it relies on data from the sources referenced.