Understanding the Risks of Model Collapse in AI Research
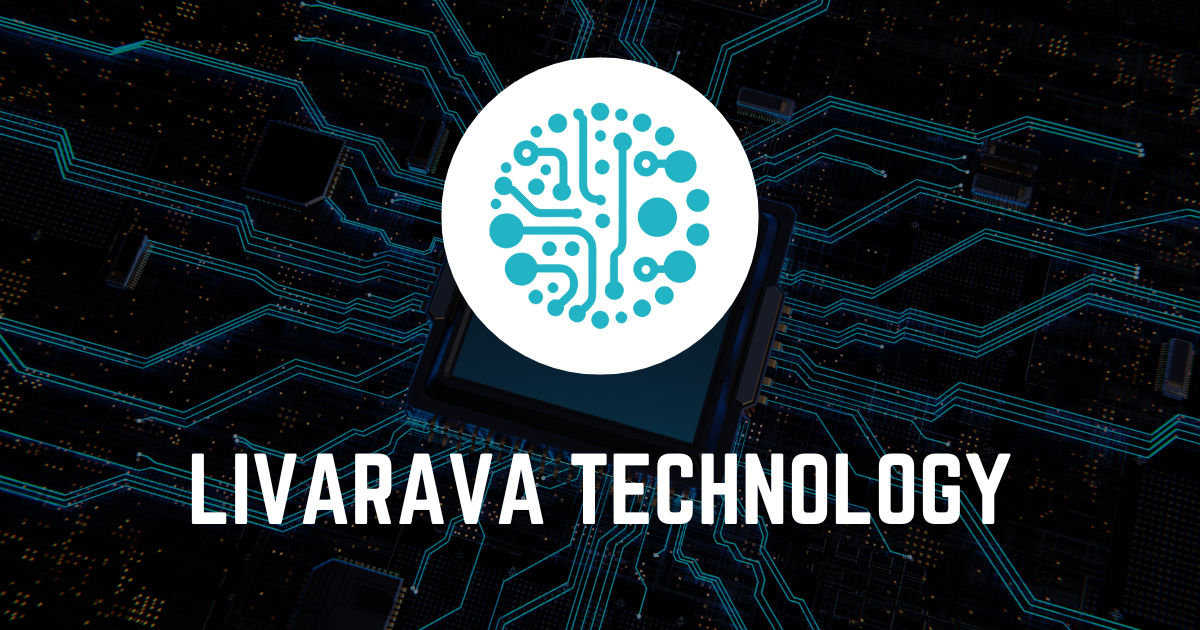
Understanding Model Collapse
AI researchers are encountering a critical issue referred to as model collapse. This phenomenon raises alarms primarily due to its impact on the effectiveness and stability of AI systems.
What Causes Model Collapse?
- Training on insufficiently diverse datasets
- Overfitting to specific problem sets
- Neglecting validation procedures
Consequences of Model Collapse
The consequences of model collapse can be detrimental, resulting in unpredictable AI behavior and diminishing trust in AI applications. As these models fail to generalize, their reliability decreases significantly.
Conclusion
Addressing the issue of model collapse is essential for the future of AI research. By focusing on better practices in training and validation, the research community can work towards sustainable and trustworthy advancements in AI technology.
This article was prepared using information from open sources in accordance with the principles of Ethical Policy. The editorial team is not responsible for absolute accuracy, as it relies on data from the sources referenced.