Understanding the Critical Role of Data Quality in Machine Learning
Monday, 5 August 2024, 06:27
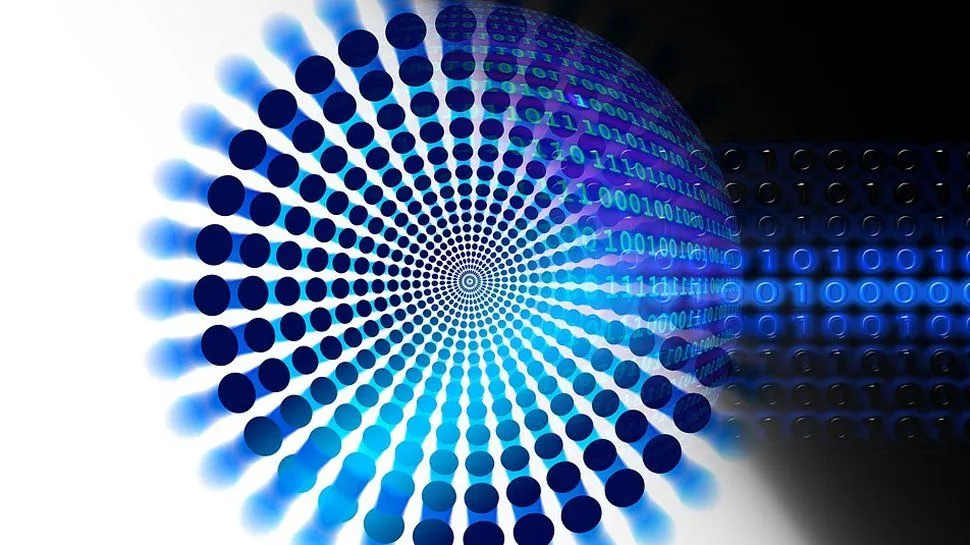
Overview of Data Quality in Machine Learning
Data quality is a crucial factor in achieving successful machine learning outcomes.
The Consequences of Poor Data Quality
- Inaccurate Models: Flawed data leads to models that do not accurately reflect the reality.
- Skewed Results: Businesses may make misguided decisions based on incorrect analyses.
- Project Failures: Lack of attention to data quality can derail initiatives.
Improving Data Quality
- Implement robust data management practices.
- Regularly audit data sources for integrity.
- Train staff on the importance of data quality.
Therefore, addressing data quality should be prioritized for organizations aiming to leverage machine learning effectively.
This article was prepared using information from open sources in accordance with the principles of Ethical Policy. The editorial team is not responsible for absolute accuracy, as it relies on data from the sources referenced.