Evaluating the Effects of Noise on Machine Learning Models in Voice Disorder Diagnosis
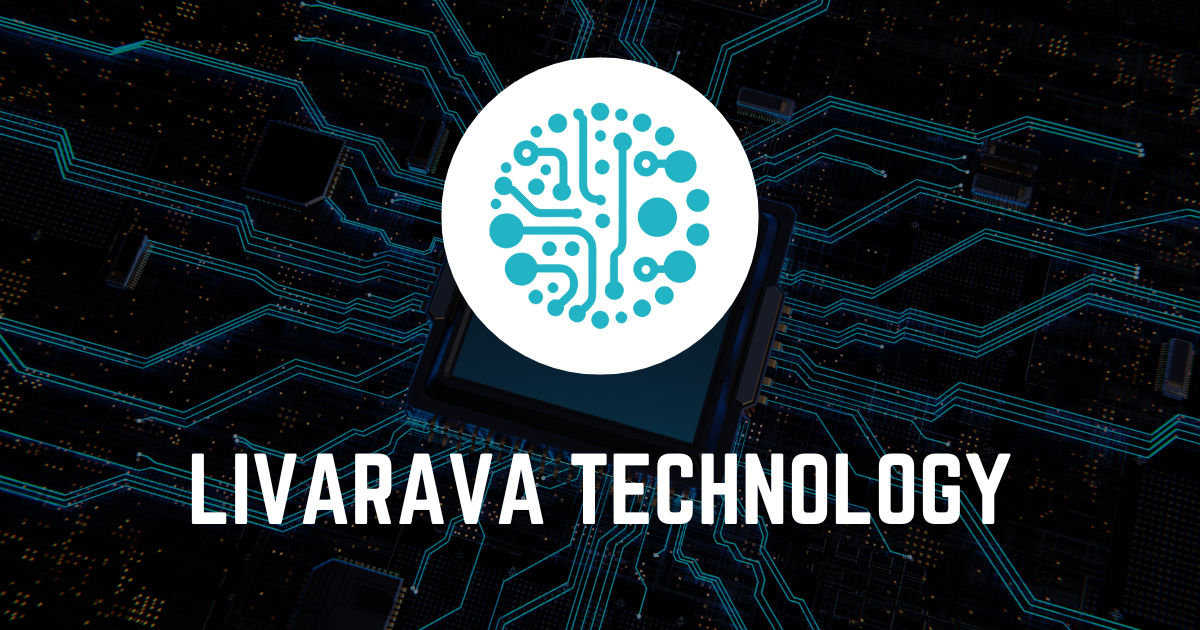
Assessing Noise Impact
In the field of voice disorder evaluation, noise can significantly alter the effectiveness of machine learning models. By identifying the types of noise that most affect these models, researchers can improve the accuracy of diagnostic tools.
Factors Influencing Performance
- Types of noise
- Data accuracy
- Model reliability
Conclusion
Addressing the impact of noise is essential for enhancing the performance of machine learning in voice disorder diagnosis, ultimately benefiting patient health outcomes.
This article was prepared using information from open sources in accordance with the principles of Ethical Policy. The editorial team is not responsible for absolute accuracy, as it relies on data from the sources referenced.