Utilizing Unsupervised Learning in the Domany-Kinzel Model for Phase Transitions
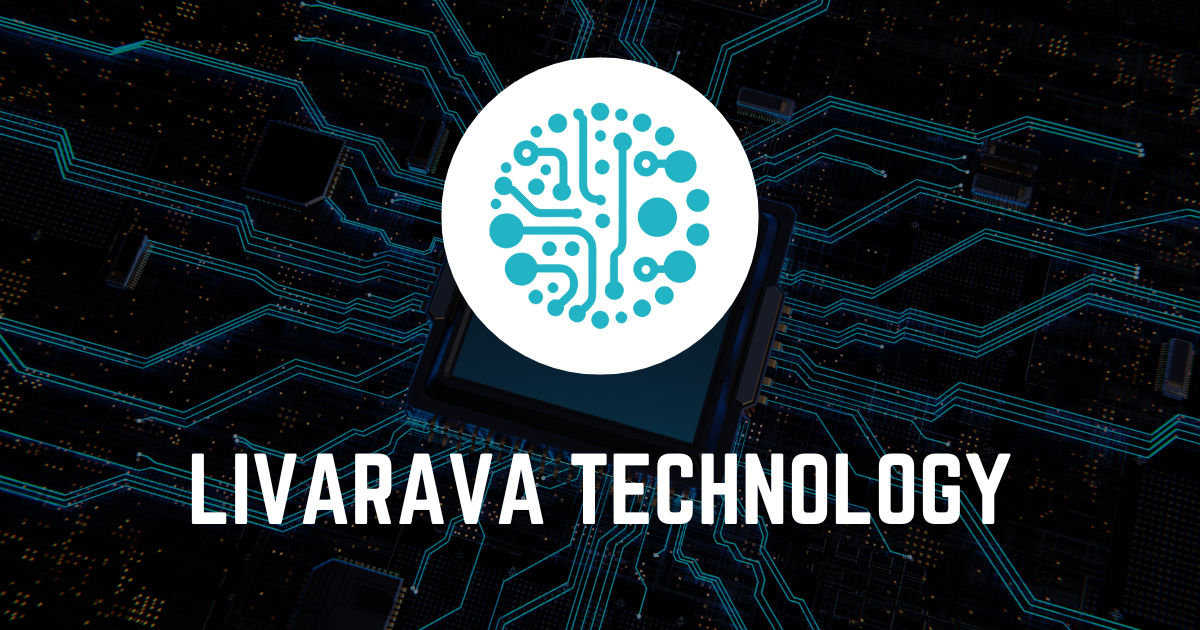
Investigating the Domany-Kinzel Model
The Domany-Kinzel (DK) model illustrates several types of nonequilibrium phase transitions based on specific parameters.
Application of Learning Methods
- Supervised learning and semi-supervised learning techniques are used to estimate critical points.
- Unsupervised methods help analyze unlabeled configurations of the DK model.
We explored the efficiency of two prominent unsupervised techniques:
- Principal Component Analysis (PCA)
- Autoencoder
Both methods have demonstrated strong correlation with simulated stationary particle number density.
Conclusion
This research highlights the potential of unsupervised learning techniques in effectively studying complex phase transitions, furthering the understanding of critical behavior in physical systems.
This article was prepared using information from open sources in accordance with the principles of Ethical Policy. The editorial team is not responsible for absolute accuracy, as it relies on data from the sources referenced.