Machine Learning Enhancements for Influenza A Virus Risk Assessment
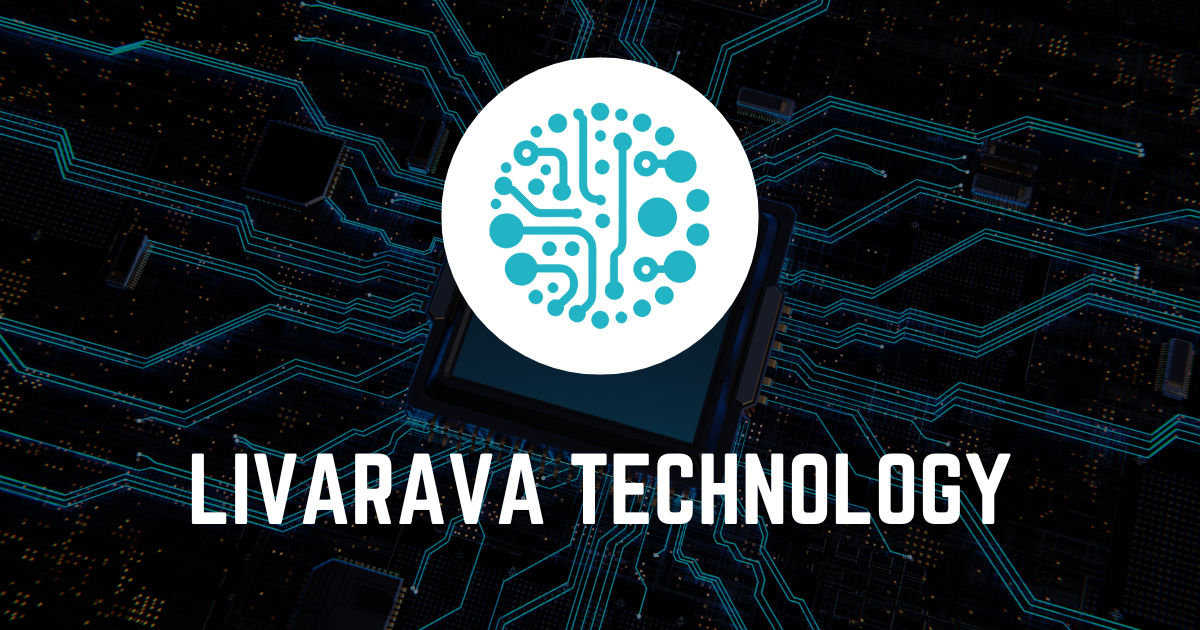
Machine Learning Approaches for Influenza A Virus
In vivo assessments of influenza A virus (IAV) pathogenicity and transmissibility in ferrets represent a crucial component of many pandemic risk assessment rubrics.
Systematic Data Aggregation
- Aggregated viral and molecular data from 125 contemporary IAV subtypes (H1, H2, H3, H5, H7, H9).
- Utilized consistent protocols for evaluation.
Predictive Classification Outcomes
- Lethality
- Morbidity
- Transmissibility
Machine learning techniques were employed to construct these outcomes based on datasets of virological and clinical parameters from inoculated ferrets.
Performance of Machine Learning Models
Among the 11 algorithms tested, gradient boosting machines and random forest algorithms yielded the highest performance, particularly in predicting lethality and transmission, outperforming models for morbidity.
Feature Selection and Validation
Comparisons of feature selection among models were performed, and the highest performing models were validated with external risk assessment studies.
Conclusion
The findings indicate that machine learning algorithms can summarize complex in vivo experimental data into actionable insights for improving pandemic preparedness criteria incorporating in vivo data.
This article was prepared using information from open sources in accordance with the principles of Ethical Policy. The editorial team is not responsible for absolute accuracy, as it relies on data from the sources referenced.