Exploring Supervised Deep Learning's Edge over Autoencoders in Propensity Score Estimation
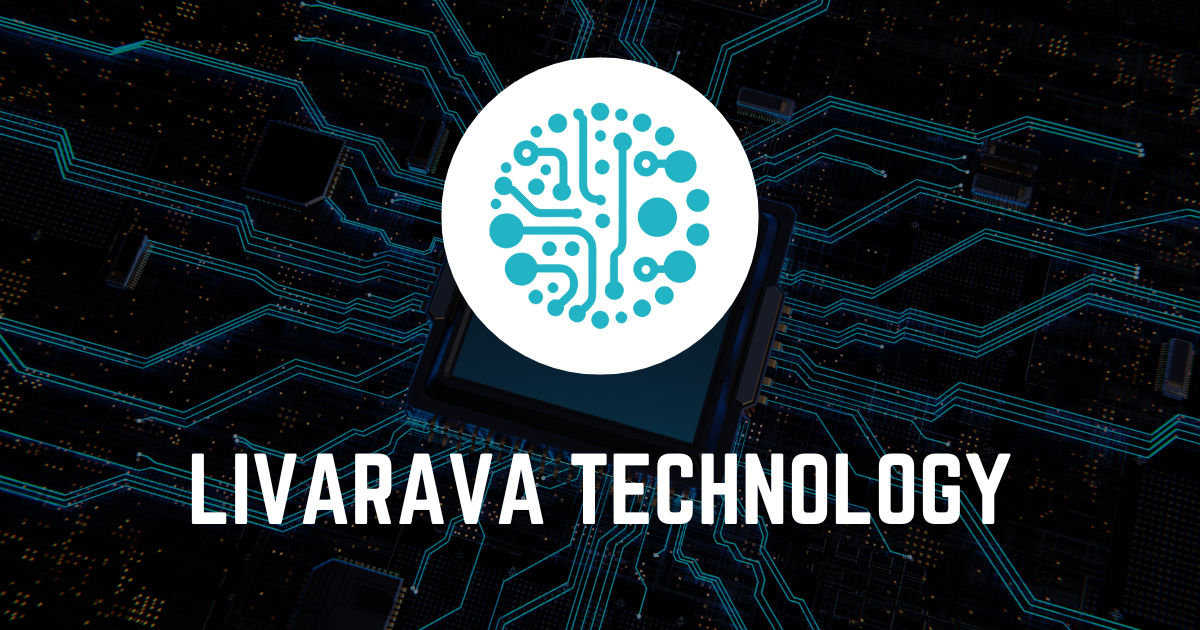
Introduction to Propensity Score Matching
Propensity score matching is crucial in epidemiological studies, leveraging observational data for accurate estimations. However, its effectiveness depends significantly on the appropriate model specification.
Study Overview
- Objective: Compare supervised deep learning models and unsupervised autoencoders for propensity score estimation.
- Methods: Application of a plasmode simulation with Right Heart Catheterization dataset.
- Comparison: Evaluated against traditional methods including logistic regression and spline-based approaches.
Key Findings
- Performance: Supervised deep learning models showed superior performance in variance estimation.
- Bias Levels: They maintained comparable bias to traditional methods.
- Real-World Data: Estimates from the supervised model closely matched conventional methods, indicating reliability.
Conclusion
These findings signify that integrating supervised deep learning into epidemiological research can significantly enhance propensity score estimations, particularly in complex datasets, thus providing improved confounder adjustments.
This article was prepared using information from open sources in accordance with the principles of Ethical Policy. The editorial team is not responsible for absolute accuracy, as it relies on data from the sources referenced.