Leveraging Machine Learning for Improved Diagnostics in Emergency Departments
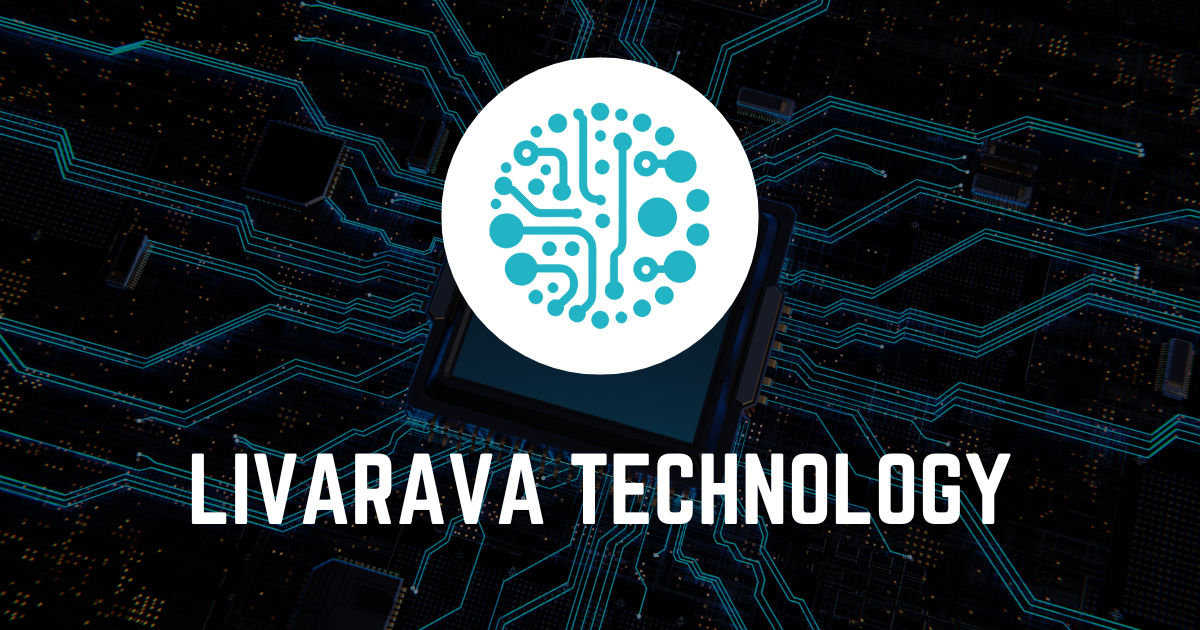
Overview of the Study
Diagnosing patients in medical emergency departments is complex and increasingly challenging due to an ageing population. This study investigates the viability of machine learning algorithms to assist physicians in these high-pressure situations.
Methods and Data
A total of 9,190 patient admissions from two hospitals were analyzed, utilizing a comprehensive biochemical workup that included 260 clinical analyses. We trained 19 machine learning algorithms on 80% of the data and validated them on the remaining 20%.
Key Findings
- AUC for main outcomes: The algorithms achieved high Area Under the Curve (AUC) scores for predicting death (91.4%) and safe discharge (87.3%).
- Reduction in venipunctures by 22% was noted, enhancing the patient experience.
This pioneering research demonstrates the potential for machine learning to not only increase diagnostic accuracy but also improve patient logistics within emergency departments.
This article was prepared using information from open sources in accordance with the principles of Ethical Policy. The editorial team is not responsible for absolute accuracy, as it relies on data from the sources referenced.