Shaila Niazi's Breakthrough in Using Probabilistic Hardware for Deep Learning
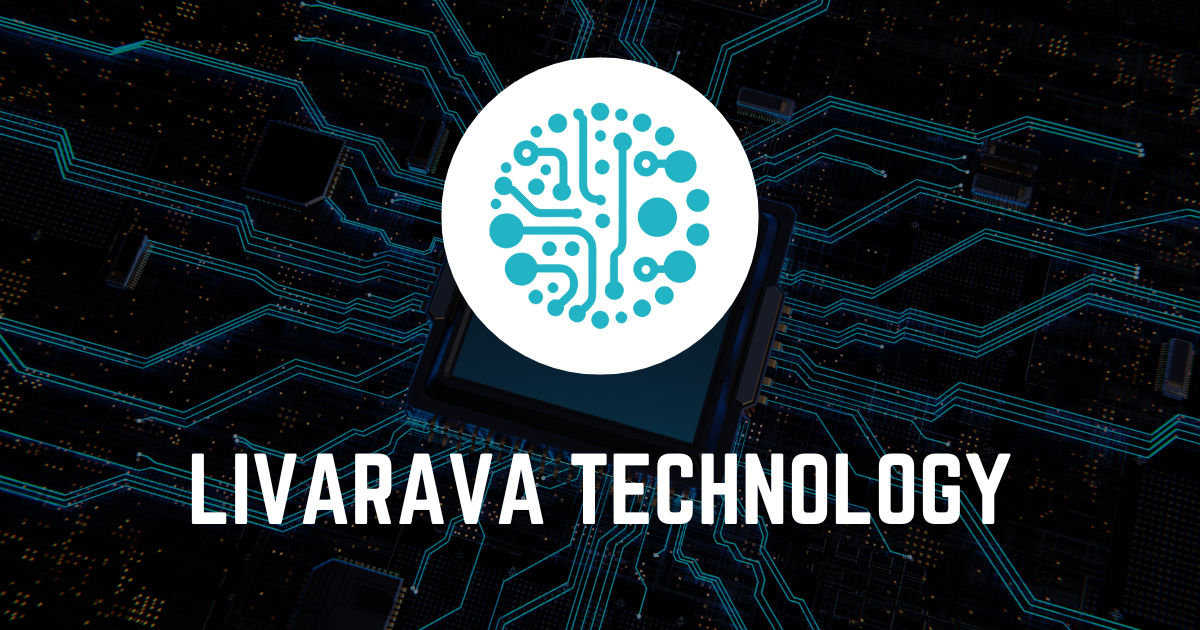
Introduction
Shaila Niazi, a third-year doctoral student, has made a significant advancement in deep learning through her use of probabilistic hardware. This innovative approach allows for the large-scale training of a deep generative model.
Innovative Approach
Niazi's research focuses on tackling real-world problems such as recognizing handwritten digits and distinguishing images of objects like birds, dogs, and cars.
Breakthrough Achievement
- First to use probabilistic hardware for deep learning at scale.
- Successfully generated images not present in the training dataset.
- Demonstrates the potential of older algorithms in modern applications.
Conclusion
This milestone in generative AI showcases the capabilities of combining traditional algorithms with innovative hardware solutions. Niazi's work opens the door for future advancements in the field.
This article was prepared using information from open sources in accordance with the principles of Ethical Policy. The editorial team is not responsible for absolute accuracy, as it relies on data from the sources referenced.