Identifying Disease Symptoms Using Machine Learning Techniques
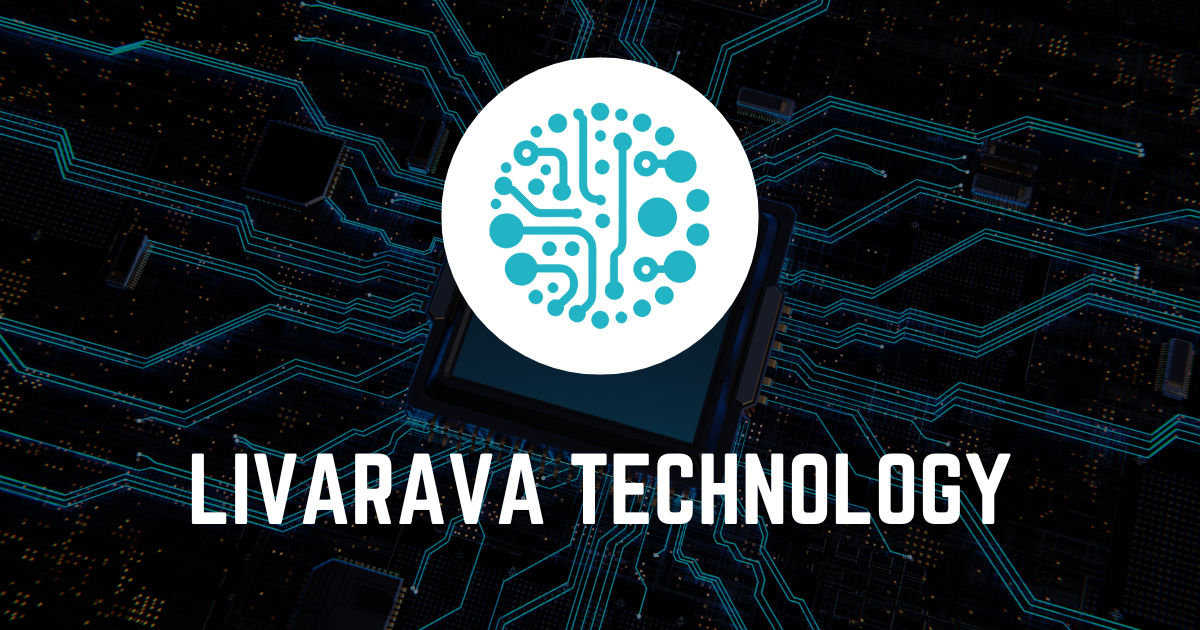
Introduction
The symptoms of diseases can vary among individuals and may remain undetected in the early stages. Detecting these symptoms is crucial in the initial stage to effectively manage and treat cases of varying severity.
Machine Learning in Healthcare
Machine learning has made major advances in recent years, proving its effectiveness in various healthcare applications. This study aims to identify patterns of symptoms and general rules regarding symptoms among patients using both supervised and unsupervised machine learning.
Analysis and Methodology
- The integration of a rule-based machine learning technique and classification methods.
- Data analysis using patient data available online through the Kaggle repository.
- Preprocessing the data and exploring descriptive statistics.
- Applying the Apriori algorithm to identify frequent symptoms and patterns.
Predictive Modeling
- Various machine learning models were employed for predicting diseases including:
- Stepwise regression
- Support Vector Machine
- Bootstrap Forest
- Boosted Trees
- Neural-boosted methods
The stepwise method for fitting outperformed all competitors, as determined through cross-validation. Numerous significant decision rules were extracted to streamline clinical applications.
Conclusion
These rules enable the prediction of relationships between symptoms and diseases, improving the performance of prediction models. This proposed algorithm has the potential to support healthcare professionals and patients facing cost and time constraints.
This article was prepared using information from open sources in accordance with the principles of Ethical Policy. The editorial team is not responsible for absolute accuracy, as it relies on data from the sources referenced.