Understanding Noise-Induced Transitions through Machine Learning
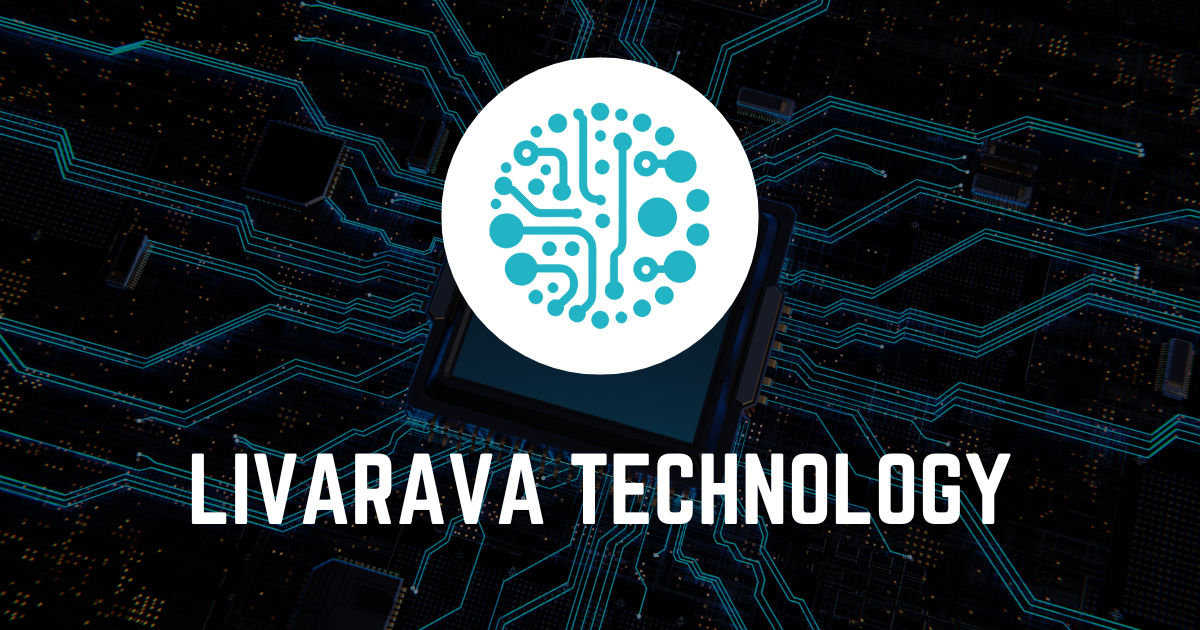
Noise's Dual Role in Stochastic Dynamics
While noise is typically viewed as a challenge in extracting clear dynamics from time series, it can also serve a functional role in driving transitions between stable states. This duality is essential for understanding complex systems.
Introducing Reservoir Computing
The authors propose leveraging reservoir computing, a machine learning approach that can effectively learn noise-induced transitions. This method stands out against conventional approaches, such as SINDy and recurrent neural networks, which often fail to capture stochastic transitions accurately.
Key Findings
- The method applies to both white noise and colored noise in bistable systems.
- Reservoir computing generates accurate statistics of transition times.
- It effectively captures the complexities of multi-stable systems and accounts for asymmetric potential.
Applications in Experimental Data
In practical terms, the approach can also analyze experimental data such as protein folding, revealing transition dynamics from even small datasets. This capability demonstrates the potential to extend existing frameworks for learning from noisy time series.
Conclusion
The findings highlight the possibility of utilizing noise as a catalyst for learning dynamics, paving the way for advancements in how we understand and model stochastic systems.
This article was prepared using information from open sources in accordance with the principles of Ethical Policy. The editorial team is not responsible for absolute accuracy, as it relies on data from the sources referenced.