Exploring the Role of Parabolic PDEs in Advancing Machine Learning Techniques
Tuesday, 30 July 2024, 07:31
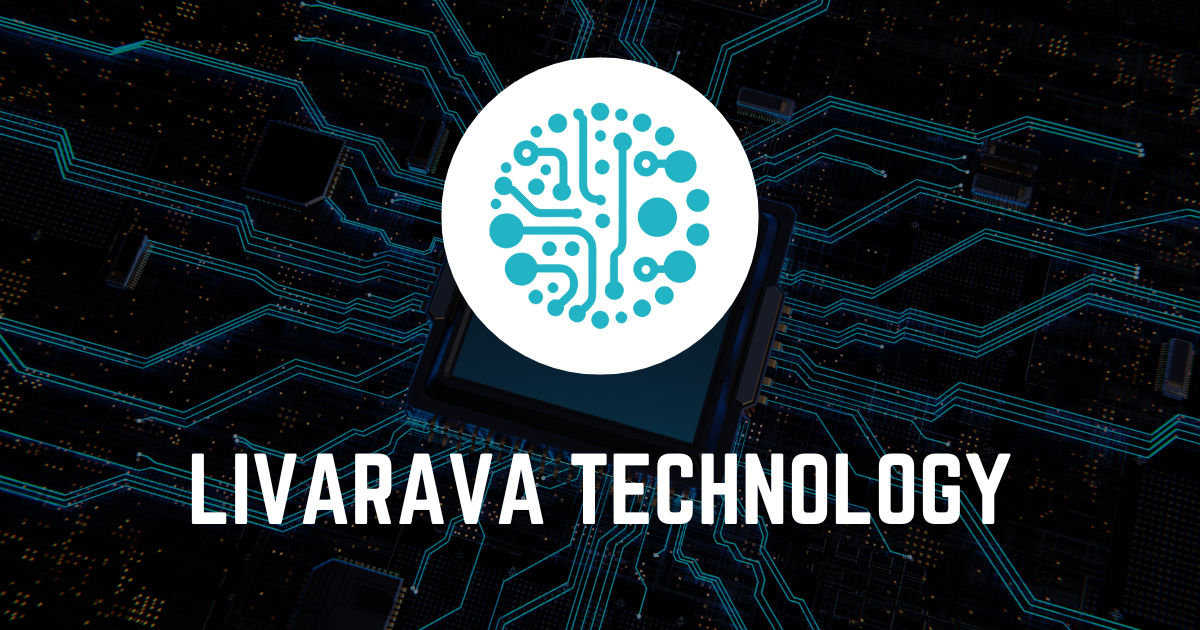
Understanding Parabolic PDEs
Parabolic Partial Differential Equations (PDEs) are crucial in various scientific fields. They describe systems that evolve over time.
Applications in Machine Learning
- Enhancement of Prediction Models: Parabolic PDEs facilitate improved accuracy in machine learning algorithms.
- Mathematical Foundation: These equations provide a rigorous framework for analyzing data.
Conclusion
The integration of Parabolic PDEs in machine learning highlights significant potential for innovation in predictive analytics.
This article was prepared using information from open sources in accordance with the principles of Ethical Policy. The editorial team is not responsible for absolute accuracy, as it relies on data from the sources referenced.