Machine Learning Revolutionizes Bottom-Jet Measurements at CERN
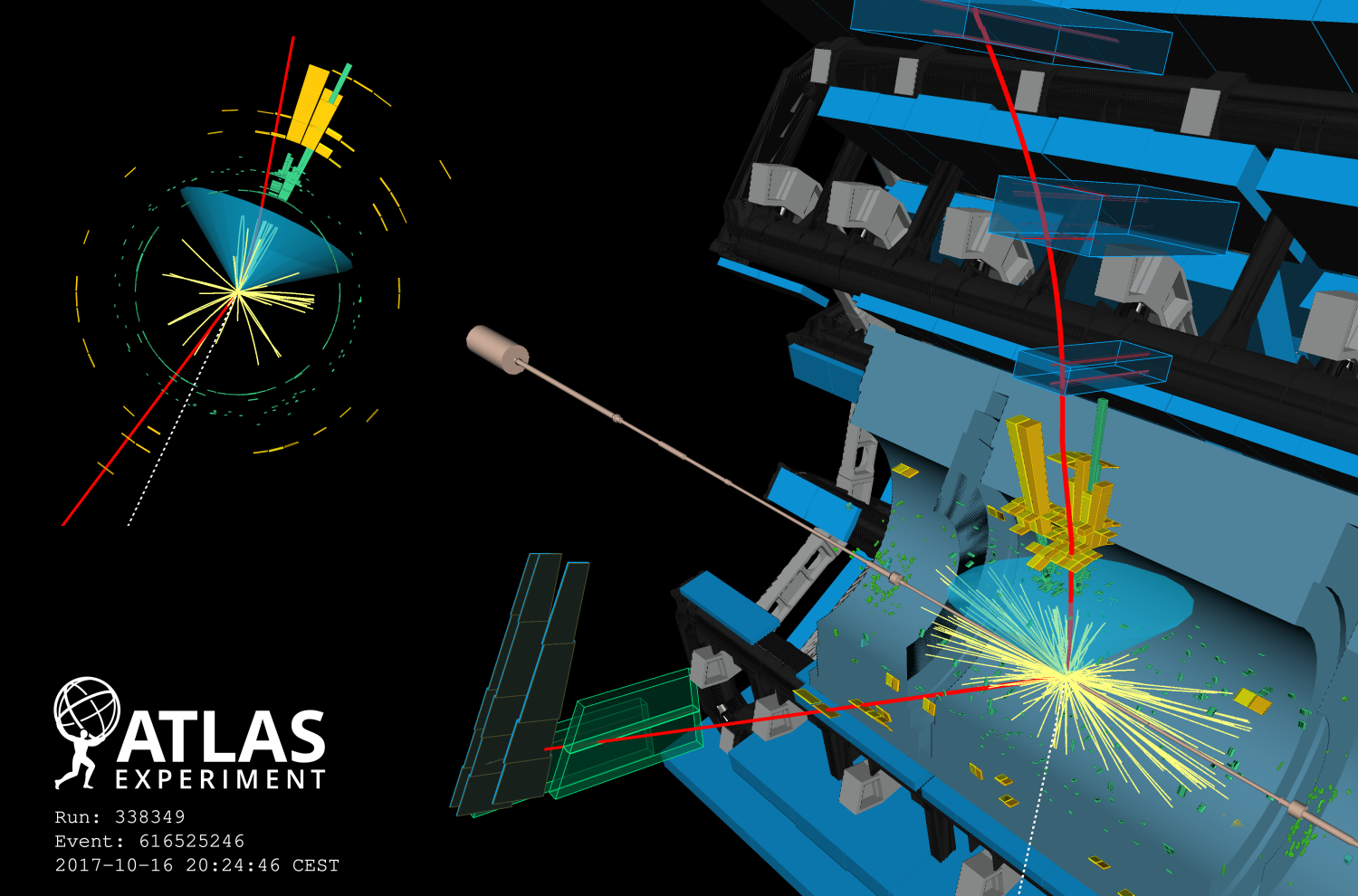
Machine Learning Enhancements in Bottom-Jet Measurements
The ATLAS Experiment at CERN is now utilizing state-of-the-art machine learning techniques for more accurate bottom-jet measurements. This technology allows for improved data collection methods, which is crucial for high-energy physics research.
Key Benefits of Machine Learning Integration
- Precision: Enhanced measurement accuracy through advanced algorithms.
- Efficiency: Faster data processing and analysis methods.
- Insights: Better understanding of particle interactions.
Conclusion
The implementation of machine learning in bottom-jet measurement techniques marks a significant advancement in scientific research abilities at CERN. These enhancements are set to revolutionize data collection and analysis in particle physics.
This article was prepared using information from open sources in accordance with the principles of Ethical Policy. The editorial team is not responsible for absolute accuracy, as it relies on data from the sources referenced.