HyPO: An Innovative Approach to Reinforcement Learning Using Hybrid Techniques
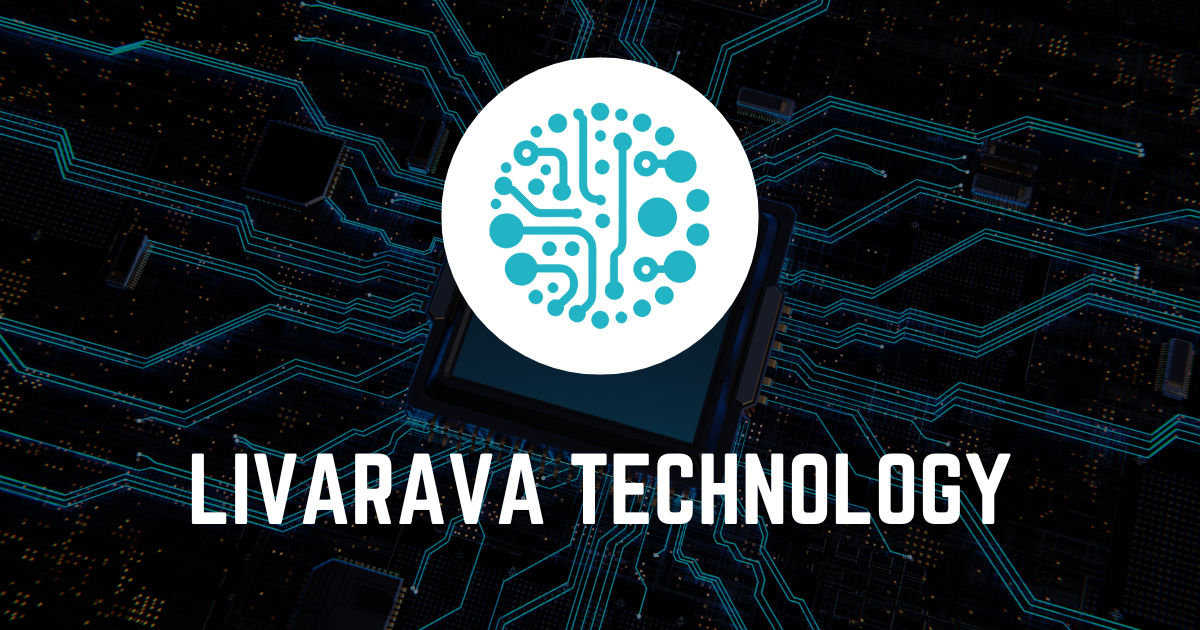
HyPO: A New Era in Reinforcement Learning
The HyPO algorithm represents a breakthrough in the application of hybrid reinforcement learning methods. By integrating offline data for contrastive-based preference optimization and utilizing online unlabeled data for KL regularization, this approach is designed to optimize learning outcomes.
Key Features of HyPO
- Contrastive-based Optimization: Increases the algorithm's ability to distinguish preferences.
- KL Regularization: Helps maintain consistency and avoids overfitting during training.
- Data Efficiency: Reduces the need for extensive labeled datasets.
Conclusion
In summary, HyPO's innovative design illustrates the evolving landscape of reinforcement learning, showcasing how hybrid methodologies can significantly improve learning processes. This development not only paves the way for future advancements in artificial intelligence but also positions HyPO as a valuable tool for practitioners in the field.
This article was prepared using information from open sources in accordance with the principles of Ethical Policy. The editorial team is not responsible for absolute accuracy, as it relies on data from the sources referenced.