Predicting Adverse Birth Outcomes in Sub-Saharan Africa with Machine Learning
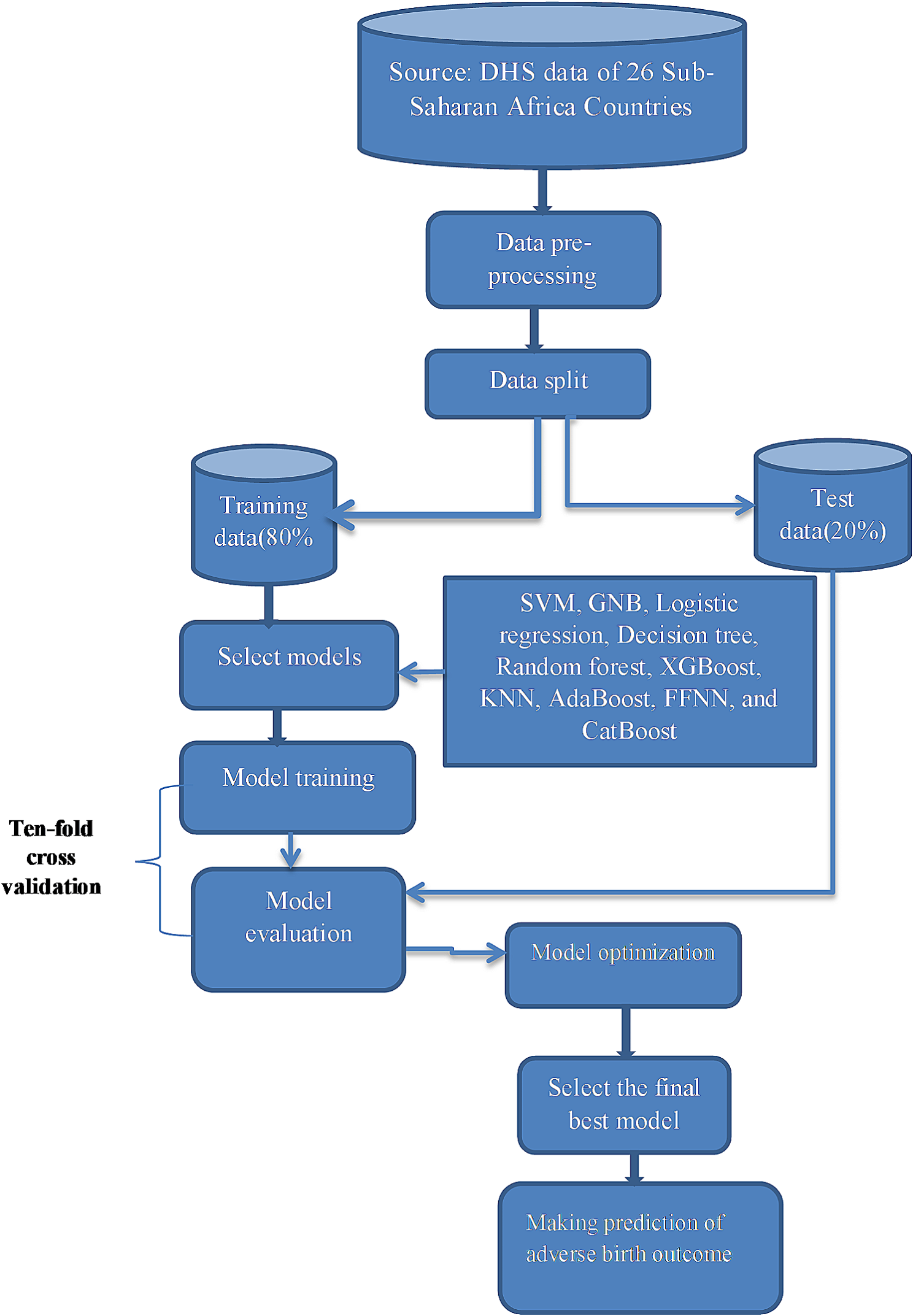
Introduction
Adverse birth outcomes, including preterm birth, low birth weight, and stillbirth, are pressing health issues in Sub-Saharan Africa.
Study Overview
A recent study aimed to develop a predictive model using innovative machine learning techniques, focusing on childbearing women in the region.
- Data was collected from women in 26 Sub-Saharan African countries.
- A total of 139,659 participants were included in the research.
Methodology
The research utilized various machine learning models, specifically:
- Data balancing techniques
- Ten advanced machine learning algorithms
- Association rule mining and SHAP analysis for interpretability
Key Findings
Results indicated that about 28.59% of women experienced adverse birth outcomes. The random forest algorithm was the top performer, achieving an AUC of 0.95 and an accuracy of 88.0%.
Identified Risk Factors
- Home deliveries
- Lack of prenatal iron supplementation
- Fewer than four antenatal care visits
- Short and long delivery intervals
- Unwanted pregnancies
- Primiparous mothers
Conclusion
The study highlights the critical need for targeted prenatal interventions in high-risk groups. Recommendations include providing iron supplements, enhancing prenatal care, and promoting skilled birth attendance.
This article was prepared using information from open sources in accordance with the principles of Ethical Policy. The editorial team is not responsible for absolute accuracy, as it relies on data from the sources referenced.