The Role of Explainable AI in Early Diagnosis of Gastric Cancer
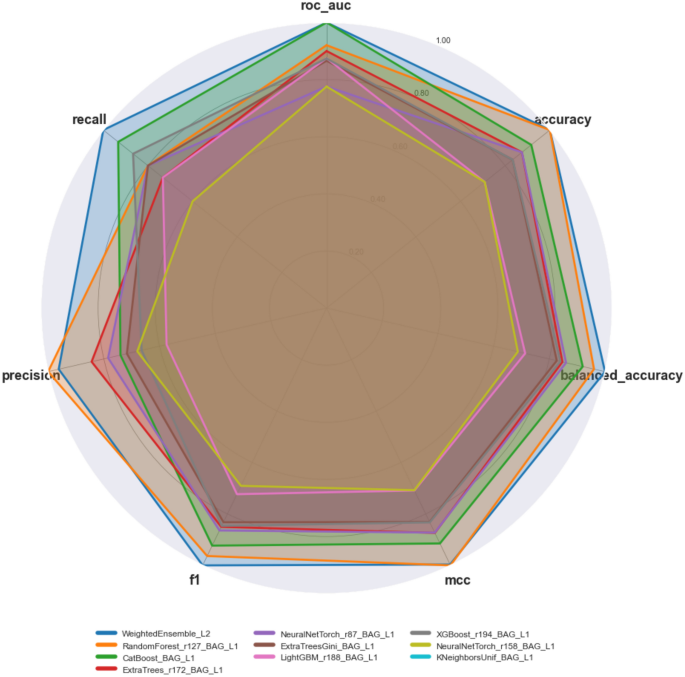
Understanding the Impact of Machine Learning in Gastric Cancer
Gastric cancer is a major global health issue, particularly prevalent in East Asia. This article discusses the potential of explainable machine learning (ML) models for early gastric cancer diagnosis.
Key Findings from the Study
- The study evaluates the performance of various ML models, including WeightedEnsemble, CatBoost, and RandomForest.
- These models showed a high capacity for accurate diagnosis of early gastric cancer.
- Model explainability is crucial, as it fosters trust and clinical acceptance among healthcare professionals.
Conclusion
The research demonstrates the potential for implementing explainable ML models in medical diagnostics to improve patient outcomes. By identifying key biomarkers and clinical features essential for early detection, this versatile approach not only advances gastric cancer diagnostics but also paves the way for greater adoption of AI technologies in the medical field.
This article was prepared using information from open sources in accordance with the principles of Ethical Policy. The editorial team is not responsible for absolute accuracy, as it relies on data from the sources referenced.