Predicting Graft and Patient Outcomes in Kidney Transplantation Using Machine Learning
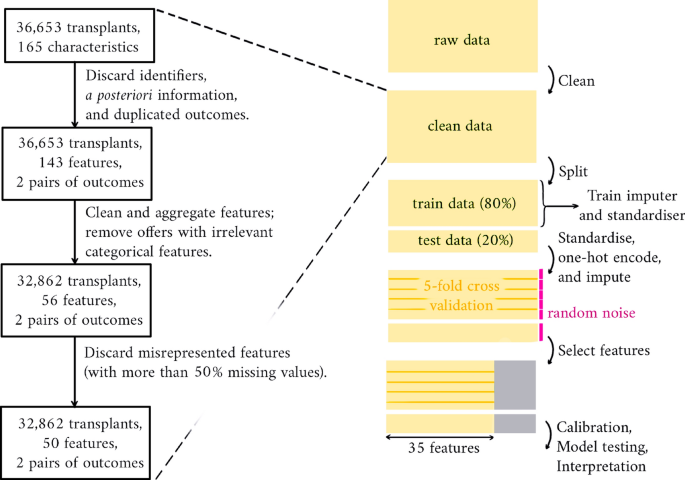
Introduction
The decision to accept a deceased donor organ for kidney transplantation can be a challenging one due to uncertain outcomes. This project addresses the need for clinical decision support tools that can predict transplant outcomes.
Research Overview
- This study employs interpretable machine learning methods to forecast graft failure and patient death.
- It uses data spanning over 25 years of transplant outcomes.
- Several survival analysis models were trained and compared.
Key Findings
- Neural networks exhibited comparable performance to the Cox proportional hazard model.
- Concordance rates achieved were 0.63 for graft failure and 0.79 for patient death.
- Important predictive features identified include donor and recipient ages, number of DR locus mismatches, dialysis type, and primary renal disease.
Conclusion
The promising results from neural networks indicate their potential as a vital component in developing future decision support systems for organ transplants, ultimately aiding healthcare professionals in making more informed decisions about patient outcomes.
This article was prepared using information from open sources in accordance with the principles of Ethical Policy. The editorial team is not responsible for absolute accuracy, as it relies on data from the sources referenced.