Understanding Carbon Nanotube Growth Through Machine Learning
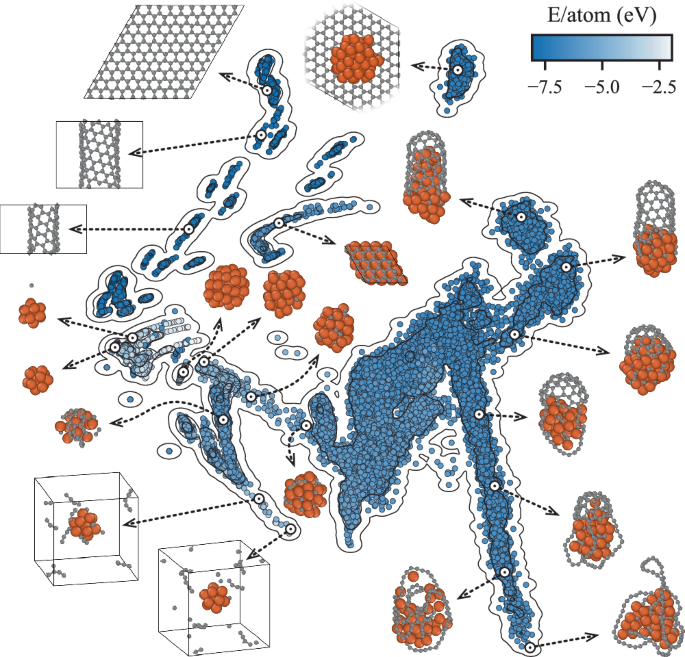
Unveiling the Dynamics of Carbon Nanotube Growth
Carbon nanotubes (CNTs) are hollow cylinders of carbon with immense potential for advanced technologies, but their properties can be compromised by structural defects. Understanding the growth process at the tube-catalyst interface is crucial, and this article explores how machine learning has transformed this insight.
DeepCNT-22: A Game Changer
The introduction of DeepCNT-22, a machine learning force field (MLFF), allows researchers to perform near-microsecond molecular dynamics simulations that illuminate the mechanisms of CNT nucleation and growth.
Key Findings
- Dynamic Interface: The tube-catalyst interface is highly dynamic, displaying significant configurational entropy.
- Defect Formation and Healing: Defects can form stochastically, but healing occurs under low growth rates and high temperatures, leading to defect-free CNTs.
- Improved Understanding: These findings provide valuable insights not easily obtainable through experimental methods.
The research highlights the transformative impact of machine learning on our understanding of carbon nanotubes, paving the way for the development of superior nanostructured materials.
This article was prepared using information from open sources in accordance with the principles of Ethical Policy. The editorial team is not responsible for absolute accuracy, as it relies on data from the sources referenced.