Exploring Neuroimaging and Natural Language Processing to Classify Suicidal Thoughts in Depression
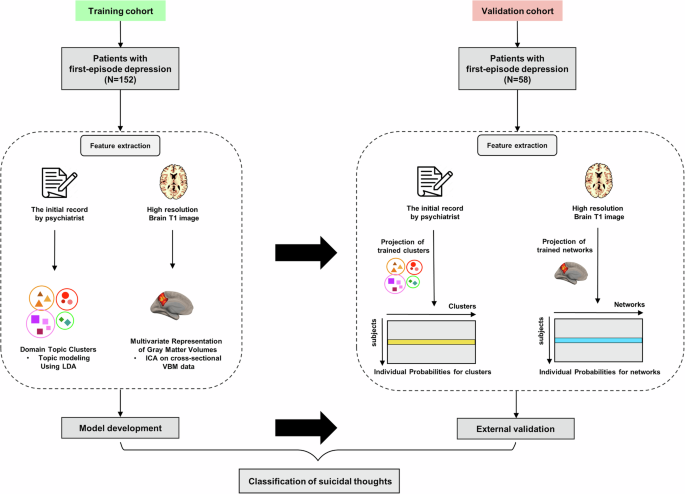
Introduction
Suicide is increasingly recognized as a critical public health issue, often linked to underlying psychiatric conditions such as depression.
Importance of Classification
Accurate classification of suicidal thoughts among depressed patients can aid in tailoring suicide prevention strategies. This study aims to address this need through machine learning techniques.
Research Methodology
- Data Sources: Unstructured psychiatric charts and brain MRI records were utilized.
- Patient Sample: The study involved 152 patients for model development and 58 for validation.
- Machine Learning Model: An eXtreme Gradient Boosting (XGBoost) model was developed, integrating neuroimaging and clinical data.
Findings
The study revealed that anxiety and somatic symptoms were significantly more prevalent in patients with suicidal ideation. Notably:
- Clinical symptoms combined with brain structure models achieved the highest accuracy (0.794).
- Neuroimaging and natural language processing showed consistent results across various metrics.
Conclusion
The results advocate for the integration of neuroimaging and language processing approaches to enhance the assessment of suicidal thoughts, potentially improving individualized treatment.
This article was prepared using information from open sources in accordance with the principles of Ethical Policy. The editorial team is not responsible for absolute accuracy, as it relies on data from the sources referenced.