Understanding the Pitfalls of Analytics and Machine Learning in Business
Monday, 15 July 2024, 07:00
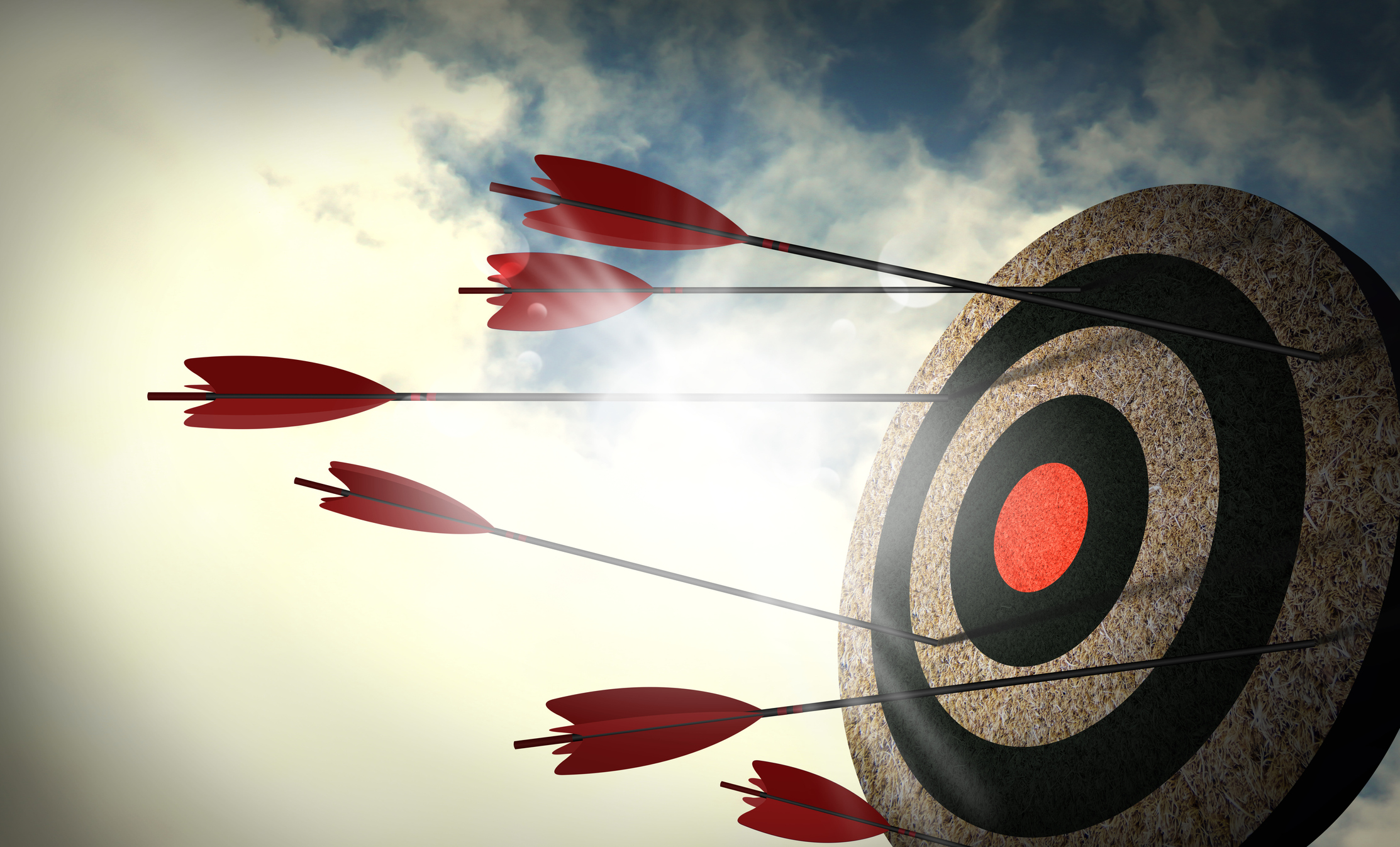
Reasons for Analytics and ML Project Failures
Data analytics and machine learning can significantly improve business outcomes. However, many projects do not meet their objectives due to several factors. Here are the top reasons for these failures:
- Poor Data Quality: Inaccurate or incomplete data leads to misguided insights.
- Lack of Clear Objectives: Without defined goals, projects lack direction.
- Insufficient Stakeholder Collaboration: Failing to involve key stakeholders results in a disconnect between project outcomes and business needs.
- Ignoring Change Management: Neglecting to plan for organizational change can hinder implementation.
- Overlooking User Experience: Complex setups or interfaces can deter usage.
- Unrealistic Expectations: Setting unattainable goals can lead to disappointment.
- Neglecting Performance Measurement: Failing to track progress makes it difficult to gauge success.
Conclusion
By systematically addressing these pitfalls, businesses can leverage analytics and machine learning more effectively, ultimately driving better results.
This article was prepared using information from open sources in accordance with the principles of Ethical Policy. The editorial team is not responsible for absolute accuracy, as it relies on data from the sources referenced.