Harnessing Machine Learning in Preclinical Drug Discovery
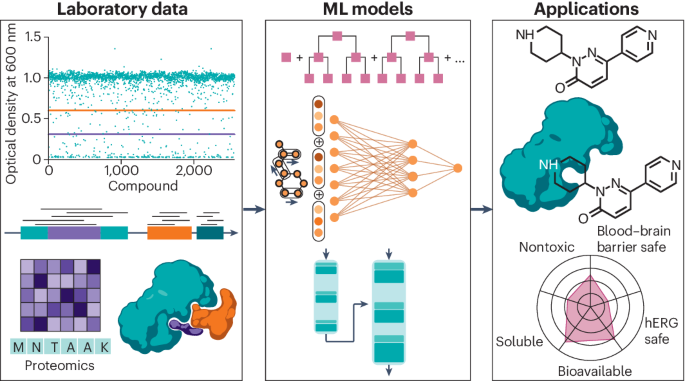
Revolutionizing Drug Discovery
Drug-discovery and drug-development endeavors are laborious, costly, and time-consuming, often exceeding 12 years and at a staggering cost of US $2.5 billion.
The Role of Machine Learning
With a failure rate of over 90%, the need for improvements in this domain is evident. Machine learning (ML) has emerged as a promising approach to enhance the drug-discovery process.
Benefits of Integrating ML
- Augmented capabilities through large-scale biological and chemical datasets.
- Accelerated initial hit discovery.
- Improved mechanism-of-action elucidation.
- Refined chemical property optimization.
Conclusion
The discussion highlights how algorithmic methods can streamline preclinical phases of drug discovery. As ML continues to evolve, it will likely define the future of drug-development programs.
This article was prepared using information from open sources in accordance with the principles of Ethical Policy. The editorial team is not responsible for absolute accuracy, as it relies on data from the sources referenced.