Modeling Chemical Processes in Explicit Solvents Using Machine Learning
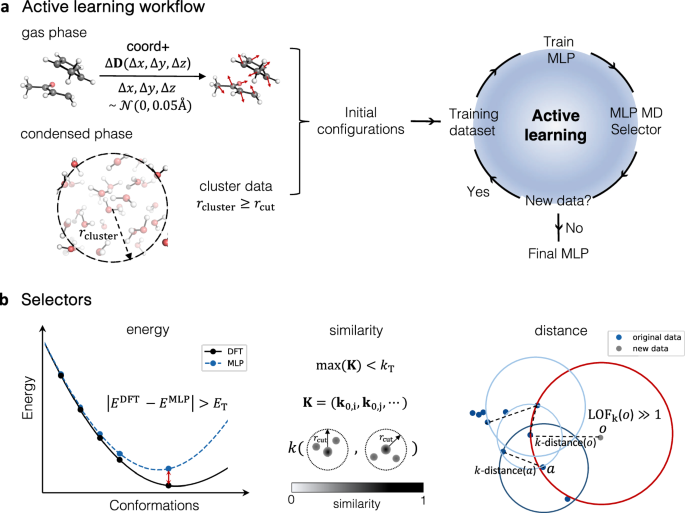
Introduction
In the field of chemistry, understanding solvent effects is crucial as they influence all stages of chemical processes. Accurately modeling these effects poses significant challenges.
Proposed Strategy
We present a general strategy for generating reactive machine learning potentials to model chemical processes in solution. This method integrates:
- Active learning
- Descriptor-based selectors
- Automation
Data Efficiency
This strategy enables the construction of data-efficient training sets that cover the relevant chemical and conformational space.
Case Study: Diels-Alder Reaction
We apply our strategy to investigate the Diels-Alder reaction in solvents like water and methanol. The results show that the generated potentials yield reaction rates that align with experimental data.
Conclusion
This automated active learning approach opens up new avenues for efficiently modeling complex chemical processes, making significant strides in the understanding of chemical reactions in solution.
This article was prepared using information from open sources in accordance with the principles of Ethical Policy. The editorial team is not responsible for absolute accuracy, as it relies on data from the sources referenced.