Harnessing Machine Learning for Advancements in the Clean-Energy Economy
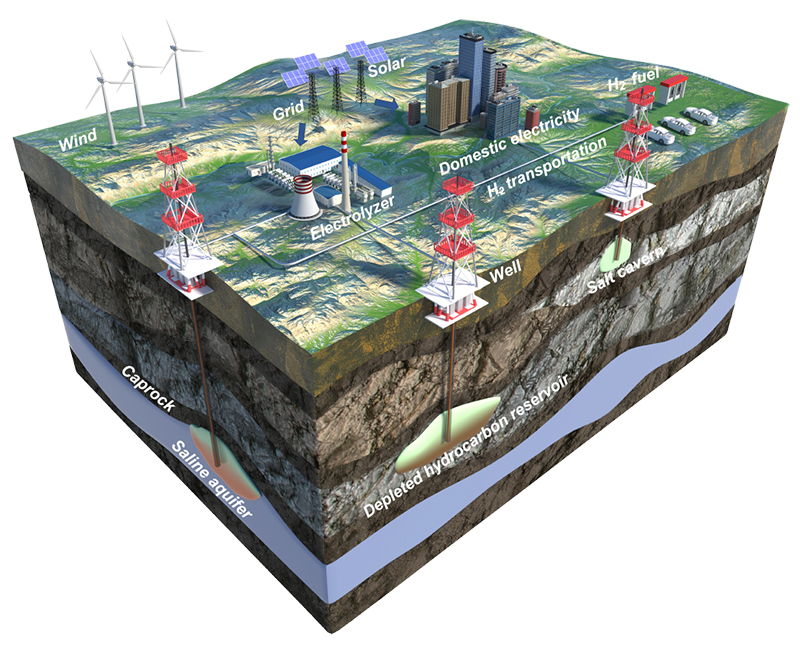
Machine Learning in Clean Energy
Scientists at Los Alamos National Laboratory (LANL) are exploring innovative uses of machine learning to improve the clean-energy sector. One of their key focuses is on underground hydrogen storage, which requires intricate operational designs.
Key Components of Underground Hydrogen Storage
- Cushion Gas: Essential for providing pressure support.
- Enhances hydrogen recovery.
- Mitigates risks related to water production.
This combination of complex operational strategies and advanced technology is critical for the advancement of the clean-energy economy, showcasing the power of artificial intelligence in modern energy solutions.
This article was prepared using information from open sources in accordance with the principles of Ethical Policy. The editorial team is not responsible for absolute accuracy, as it relies on data from the sources referenced.