Understanding In-Context Learning and Its Benefits for Large Language Models
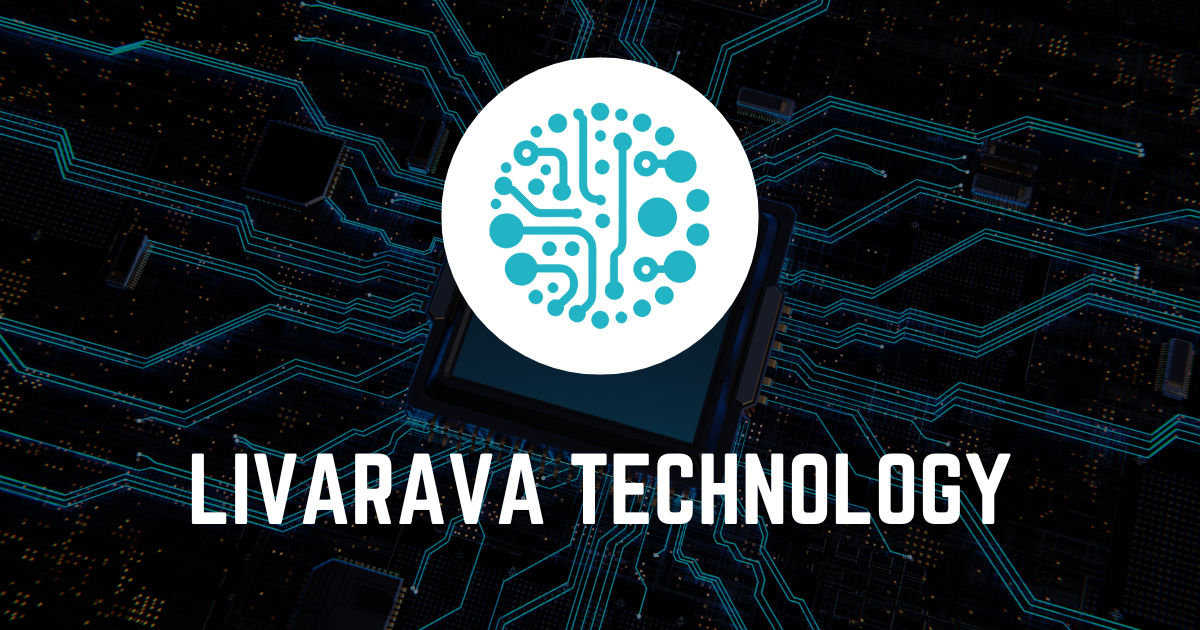
Introduction
A team at IBM Research and Rensselaer Polytechnic Institute (RPI) has unveiled important insights about in-context learning and its role in enhancing large language models.
What is In-Context Learning?
In-context learning refers to the ability of AI models to utilize contextual information to make better predictions.
Key Findings
- Improved Accuracy: The research demonstrates that in-context learning significantly boosts the predictions made by foundation models.
- Increased Transparency: This study offers deeper insights into how machine learning works, making models more interpretable.
- Future Implications: The findings could lead to improved methodologies in AI training, impacting various technology sectors.
Conclusion
The research from IBM and RPI underscores the value of in-context learning in refining the capabilities of large language models. As AI technologies continue to evolve, such breakthroughs will be vital in achieving greater transparency and effectiveness in machine learning applications.
This article was prepared using information from open sources in accordance with the principles of Ethical Policy. The editorial team is not responsible for absolute accuracy, as it relies on data from the sources referenced.