Understanding the Risks of Model Collapse in AI Training
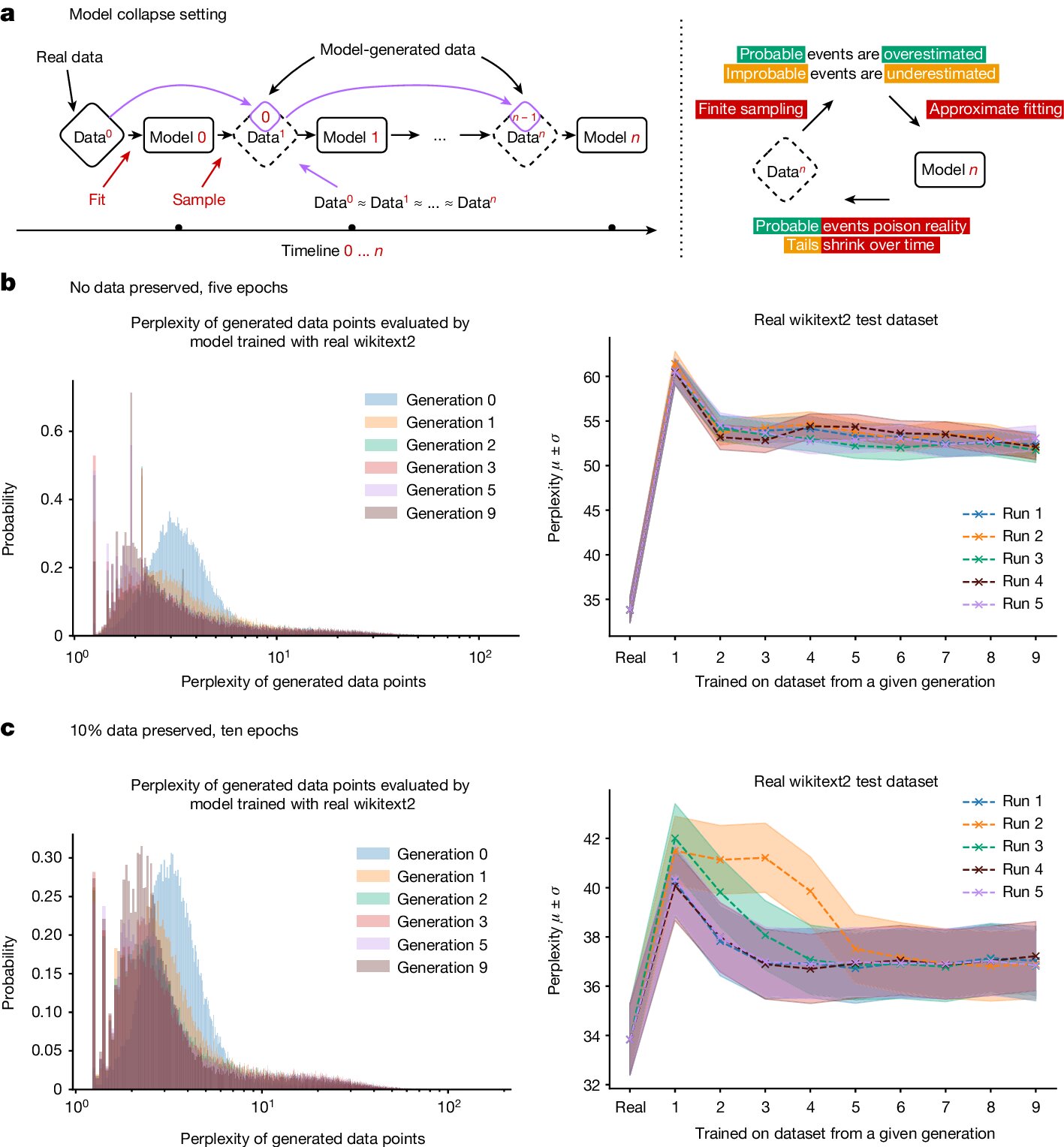
Introduction to Model Collapse
Using AI-generated datasets for training future generations of machine learning models poses significant risks, particularly the concept known as model collapse. This risk was examined in a recent paper published in Nature.
The Research Findings
Researchers demonstrated that employing AI to produce training data could potentially lead to the pollution of model outputs. They created simulations showing that models trained on biased datasets could amplify rather than mitigate existing biases.
Implications for Future AI Models
- Quality of Data: The integrity of training data is paramount for developing reliable models.
- Continuous Monitoring: Ongoing vigilance in dataset curation is necessary to prevent model collapse.
- Future Research Directions: Further investigation is required to develop more robust training methodologies.
Conclusion
This research serves as a wake-up call for developers and researchers to scrutinize their training datasets closely to avoid the pitfalls associated with model collapse. As AI technology advances, ensuring data quality will be essential to maintain the efficacy of AI systems.
This article was prepared using information from open sources in accordance with the principles of Ethical Policy. The editorial team is not responsible for absolute accuracy, as it relies on data from the sources referenced.