Data-Centric Machine Learning: A Breakthrough in Glioma Grade Prediction
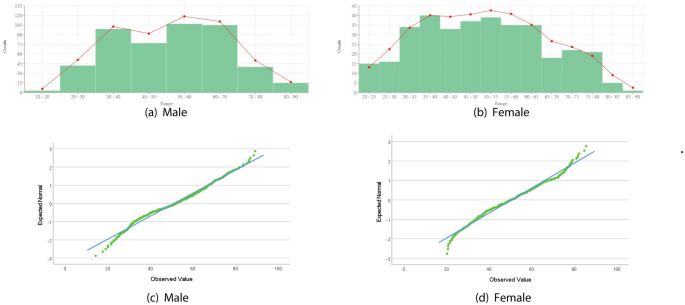
Introduction
Accurate prediction and grading of gliomas play a crucial role in evaluating brain tumor progression.
The Role of Machine Learning
In addition to neuroimaging techniques, identifying molecular biomarkers that guide diagnosis and treatment has gained interest.
Focus on Data-Centric Approaches
- This study investigates the benefits of a data-centric machine learning approach.
- Improving data quality can lead to better model performance.
Experimental Results
We report six performance metrics that provide insights into model effectiveness. The study demonstrates:
- Standardization and oversampling of the minority class improve prediction accuracy.
- Classifier ensembles significantly outperform standard prediction models.
Conclusion
This comprehensive analysis indicates the necessity of data-centric strategies in machine learning, particularly for challenging tasks like glioma grade prediction.
This article was prepared using information from open sources in accordance with the principles of Ethical Policy. The editorial team is not responsible for absolute accuracy, as it relies on data from the sources referenced.