Advanced Deep Learning Techniques for Accurate Agricultural Price Predictions in India
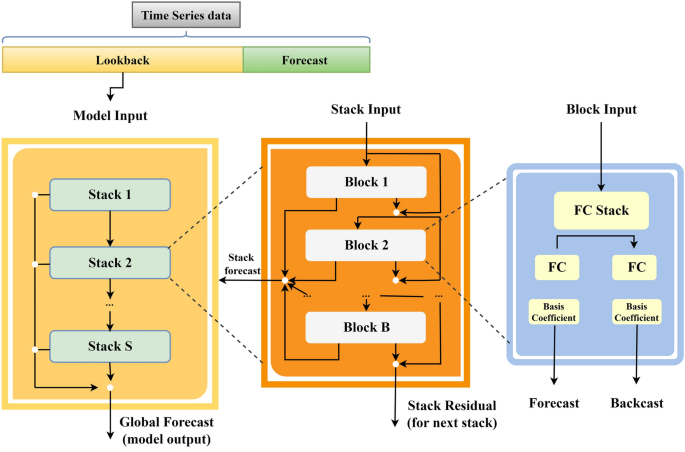
Introduction
Accurately predicting agricultural commodity prices is crucial for India's economy. Traditional parametric models struggle with stringent assumptions, while machine learning (ML) approaches, though data-driven, lack automatic feature extraction.
Deep Learning Models
Deep learning (DL) models, with advanced feature extraction and predictive abilities, offer a promising solution. This study explored advanced versions of the well-known univariate models, NBEATSX and TransformerX.
Research Methodology
- Utilized price data for essential crops like Tomato, Onion, and Potato (TOP) from major Indian markets.
- Complemented with corresponding weather data (precipitation and temperature).
- Compared performance with traditional statistical methods (ARIMAX and MLR) and various ML algorithms (ANN, SVR, RFR, and XGBoost).
Performance Evaluation
The performance was rigorously evaluated using error metrics including:
- RMSE
- MAE
- sMAPE
- MASE
- QL
Findings
The findings indicate that DL models, particularly when augmented with exogenous variables, consistently outshone other methods. NBEATSX and TransformerX showcased average RMSE of 110.33 and 135.33, respectively. They demonstrated lower error metrics compared to both statistical and ML models, underscoring their effectiveness and potential in agricultural crop price forecasting.
Conclusion
This study bridged a crucial research gap and highlighted the robust potential of DL models in enhancing the accuracy of agricultural commodity price predictions in India.
This article was prepared using information from open sources in accordance with the principles of Ethical Policy. The editorial team is not responsible for absolute accuracy, as it relies on data from the sources referenced.