Nvidia H100 GPU and HBM3 Memory Issues Impact LLama 3 Training at Meta
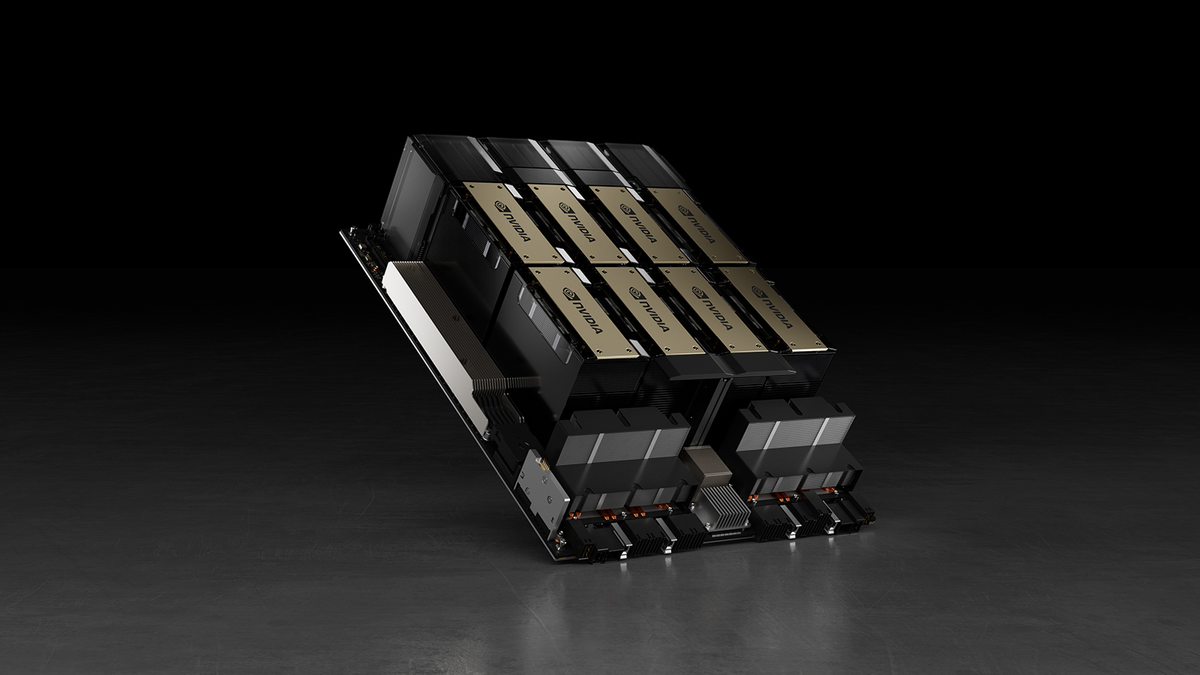
Introduction
In a large-scale training environment, hardware reliability is crucial. Recently, Meta has faced significant challenges with its 16,384 H100 GPU cluster.
Failure Rates and Causes
- Frequent Failures: Meta experiences one failure every three hours during LLama 3 training.
- H100 GPUs: These GPUs are often the main culprits behind the interruptions.
- HBM3 Memory: Issues with HBM3 memory have also contributed to the failures.
Consequences for Meta
This stream of failures not only delays progress on the LLama 3 model but also poses questions about the overall reliability of Nvidia's hardware in demanding tasks.
Conclusion
The challenges faced by Meta's training process highlight the critical importance of reliable hardware in AI development. Addressing these GPU and memory issues will be essential for ensuring future successes in high-performance computing.
This article was prepared using information from open sources in accordance with the principles of Ethical Policy. The editorial team is not responsible for absolute accuracy, as it relies on data from the sources referenced.