DeepMind's Novel Approach to Deciphering Large Language Models with Sparse Autoencoders
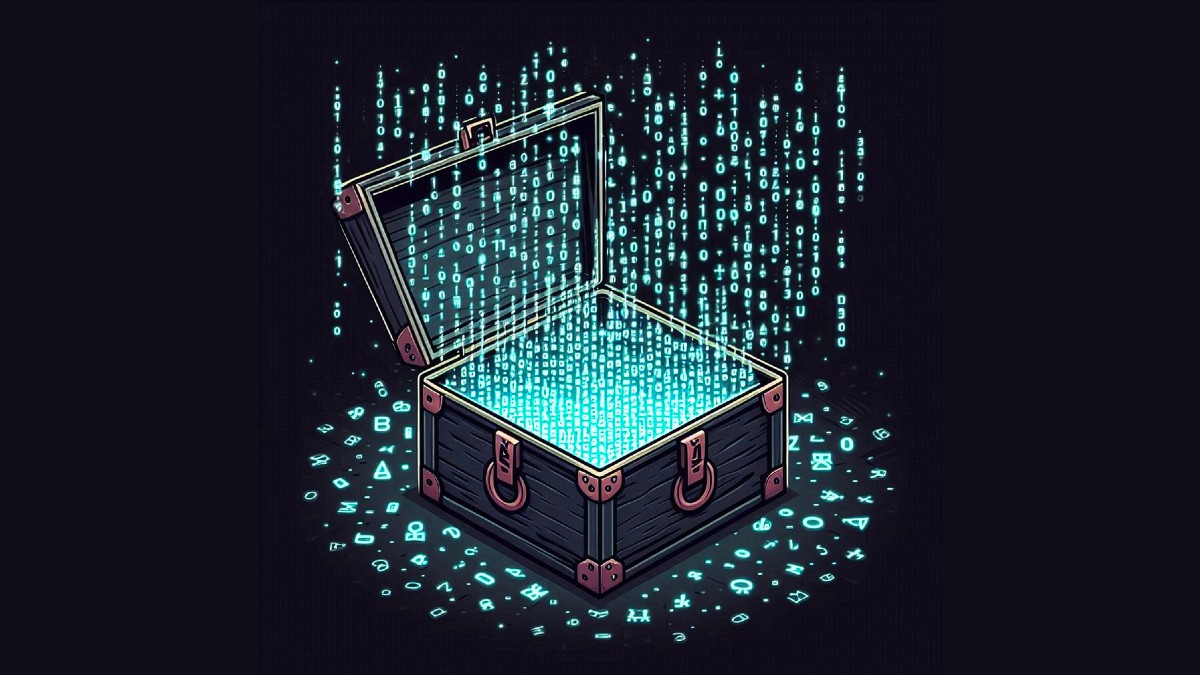
Understanding Sparse Autoencoders
Google DeepMind's latest research focuses on the use of sparse autoencoders (SAEs) to interpret the complex behaviors of large language models (LLMs). These models have become integral in various AI applications, yet their inner workings often remain opaque.
The Role of JumpReLU Activation
By integrating a unique activation function known as JumpReLU with SAEs, researchers aim to clarify how LLMs process information. This combination shows promising results in enhancing model interpretability and performance.
Implications for the Future
- Improved Model Insights: The ability to interpret LLMs can lead to better understanding and trust in AI systems.
- Broader Applications: Insights gained could be vital for diverse AI applications, from chatbots to content generation.
In conclusion, the advancement of using sparse autoencoders with JumpReLU marks a significant step forward in AI, showcasing how interpretation can drive further developments in machine learning technologies.
This article was prepared using information from open sources in accordance with the principles of Ethical Policy. The editorial team is not responsible for absolute accuracy, as it relies on data from the sources referenced.