Exploring the Role of AI and Machine Learning in Metabolomics and Exposomics
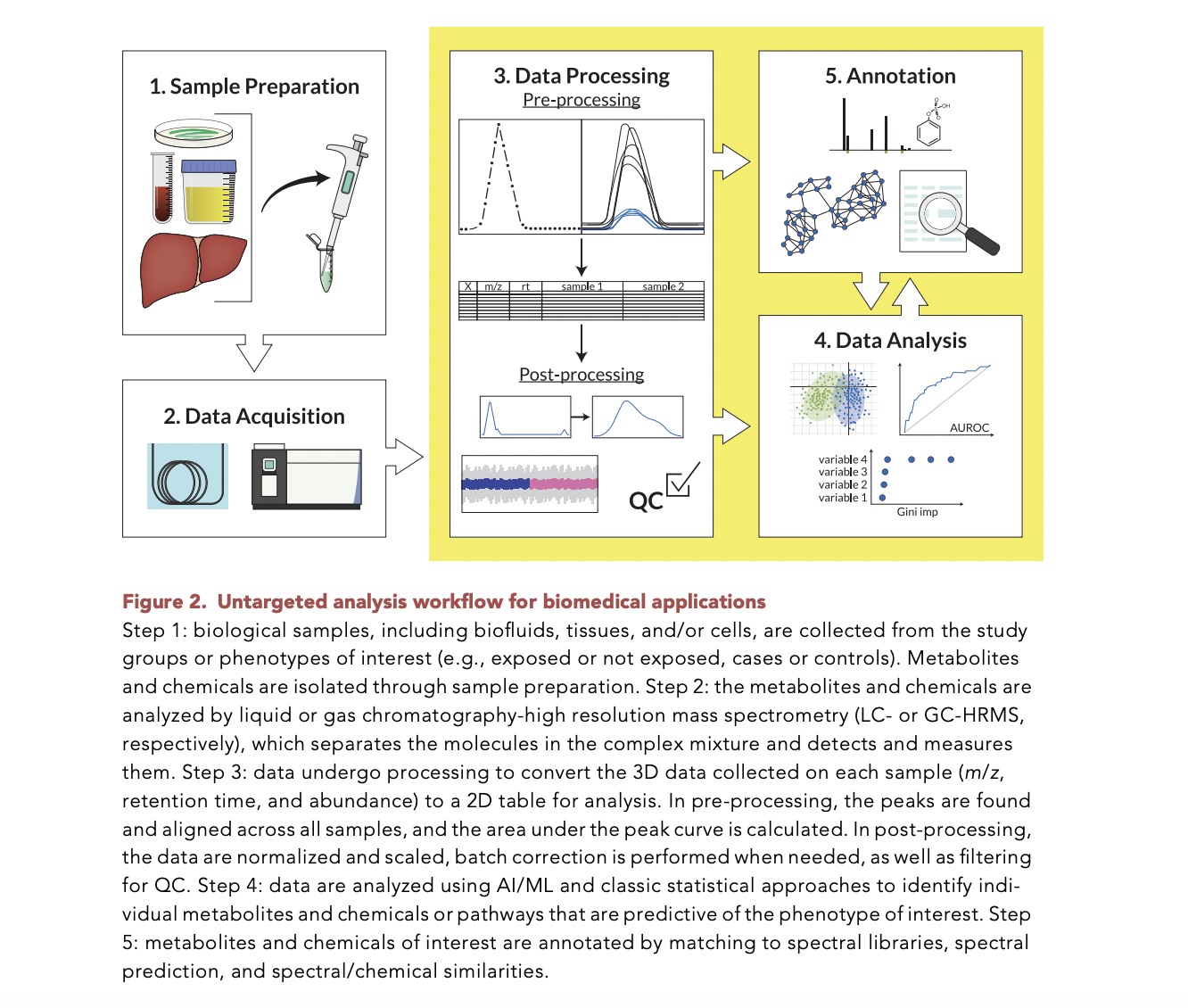
Introduction to AI and ML in Metabolomics and Exposomics
This article discusses the significant roles of artificial intelligence (AI) and machine learning (ML) in advancing untargeted metabolomics and exposomics. As these fields evolve, AI and ML are proving to be crucial tools for data analysis and interpretation.
Advancements in the Field
- Improved data processing capabilities.
- Enhanced predictive modeling.
- Integration of massive datasets.
Challenges Encountered
- Data heterogeneity.
- Interpretation complexity.
- Need for standardized methodologies.
Future Directions
Moving forward, it is essential to foster collaborations across disciplines to further enhance the contributions of AI and ML to metabolomics and exposomics. This will include:
- Expanding computational frameworks.
- Improving algorithm accuracy.
Conclusion
The integration of AI and ML into untargeted metabolomics and exposomics represents a revolutionary shift in how we analyze and understand biological and environmental data.
This article was prepared using information from open sources in accordance with the principles of Ethical Policy. The editorial team is not responsible for absolute accuracy, as it relies on data from the sources referenced.