Machine Learning Techniques to Predict Readmissions After Bariatric Surgery
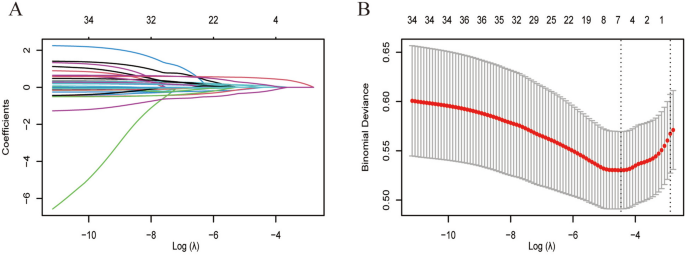
Introduction
The purpose of the study was to develop a machine learning model for predicting 30-day readmission after bariatric surgery based on laboratory tests.
Data Collection
Data were collected from patients who underwent *bariatric surgery* between 2018 and 2023. Laboratory test indicators from the preoperative stage, one day postoperatively, and three days postoperatively were analyzed.
Model Selection
Least absolute shrinkage and selection operator regression was used to select the most relevant features. Models constructed in the study included:
- Support Vector Machine (SVM)
- Generalized Linear Model
- Multi-layer Perceptron
- Random Forest
- Extreme Gradient Boosting
Model Performance
Model performance was evaluated and compared using the area under the receiver operating characteristic curve (AUROC). A total of 1262 patients were included, with 7.69% of cases being readmitted.
Results
The SVM model achieved the highest AUROC value of 0.784 (95% CI 0.696–0.872), outperforming other models. This suggests that machine learning models based on laboratory test data can effectively identify patients at high risk of readmission after bariatric surgery.
This article was prepared using information from open sources in accordance with the principles of Ethical Policy. The editorial team is not responsible for absolute accuracy, as it relies on data from the sources referenced.