Classifying Stars Using Machine Learning: Insights from LAMOST Spectra
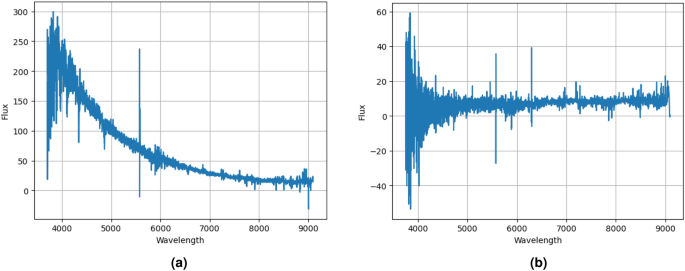
Introduction
Machine learning has emerged as a leading field in artificial intelligence, demonstrating expert-level performance in various domains.
Astronomy and Machine Learning
Astronomy has benefited from machine learning techniques, particularly in classifying and identifying stars based on their features. This study focuses on the spectra-based classification of 11,408 B-type and 2,422 hot subdwarf stars.
Methodology
- The study employs baseline correction using Asymmetric Least Squares (ALS) to enhance classification accuracy.
- It applies the Pan-Core concept to identify 500 unique patterns for both types of stars.
- These patterns form the foundation for creating Support Vector Machine (SVM) models.
Results
Parameter tuning for the SVM models is achieved through cross-validation. The evaluation of the SVM models on test data reveals that:
- Linear kernel SVM achieves the highest accuracy (87.0%)
- Polynomial kernel SVM follows with 84.1%
- Radial kernel SVM shows 80.1% accuracy.
The average calibrated accuracy falls within the range of 90–95%, demonstrating the potential of using spectrum-based classification to aid astronomers.
Conclusion
This study presents a valuable investigation for astronomers, as it enables the classification of stars based on their spectra, leveraging machine learning techniques to enhance their knowledge and insights in astronomy.
This article was prepared using information from open sources in accordance with the principles of Ethical Policy. The editorial team is not responsible for absolute accuracy, as it relies on data from the sources referenced.