Advanced Explainable Machine Learning Reveals Insights into Complex Pollutant Dynamics
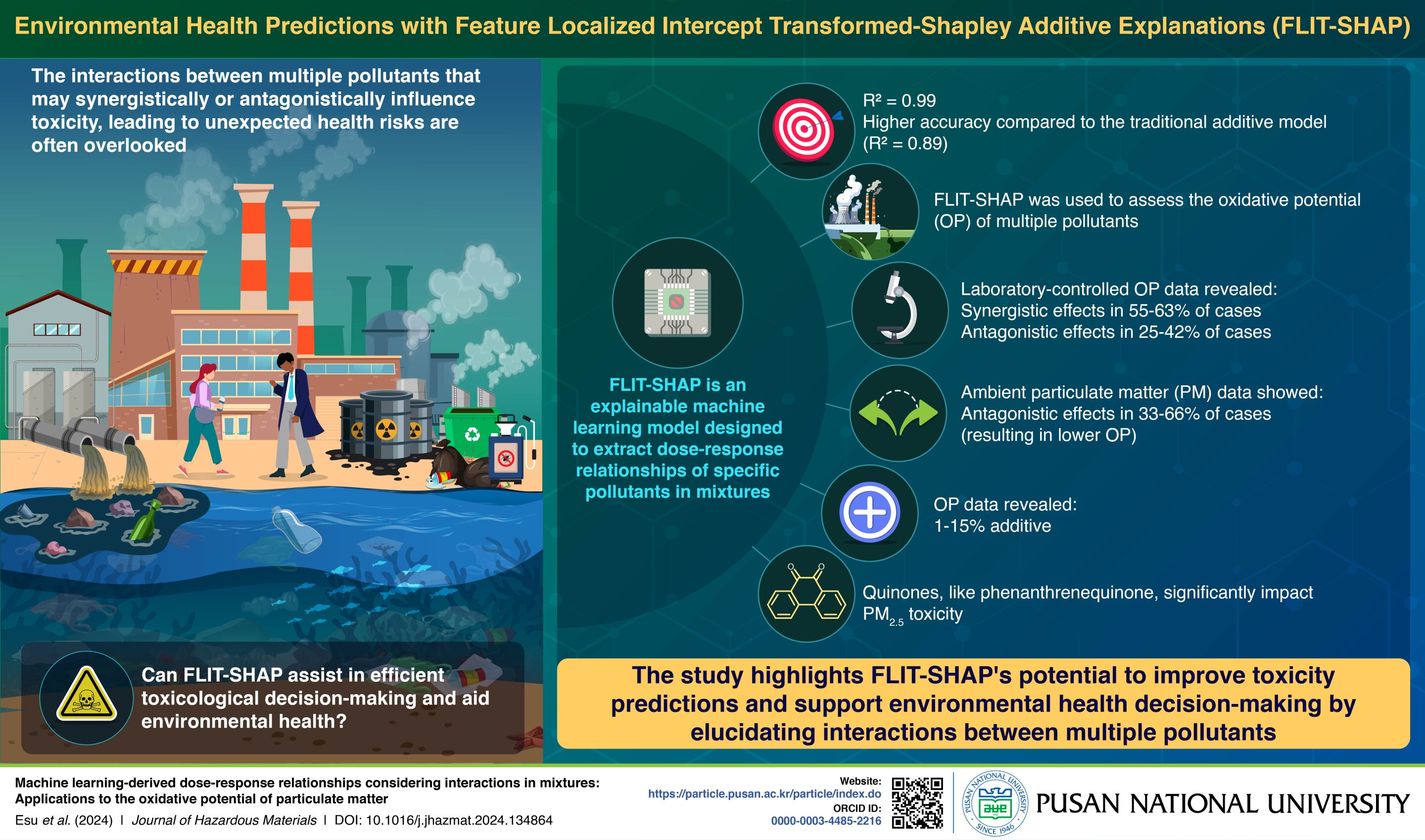
Introduction
Traditional environmental health research often focuses on the toxicity of single chemical exposures. However, in real-world situations, people are exposed to multiple pollutants simultaneously, which can interact in complex ways, potentially amplifying or diminishing their toxic effects.
Complex Interactions of Pollutants
- Multiple chemicals may combine to alter toxicity.
- Predictive models help to assess real-world exposures.
- Machine learning enables better insights into interactions.
Importance of Advanced Machine Learning
- Provides a framework for understanding complex interactions.
- Informs better public health guidelines.
- Facilitates more accurate assessments of environmental risks.
Conclusion
Utilizing advanced explainable machine learning techniques can transform the way researchers analyze pollutant interactions. This approach not only enhances our understanding of toxic effects but also underscores the necessity for comprehensive environmental assessments in public health.
This article was prepared using information from open sources in accordance with the principles of Ethical Policy. The editorial team is not responsible for absolute accuracy, as it relies on data from the sources referenced.