Exploring the Limitations of Machine Learning in Astronomy
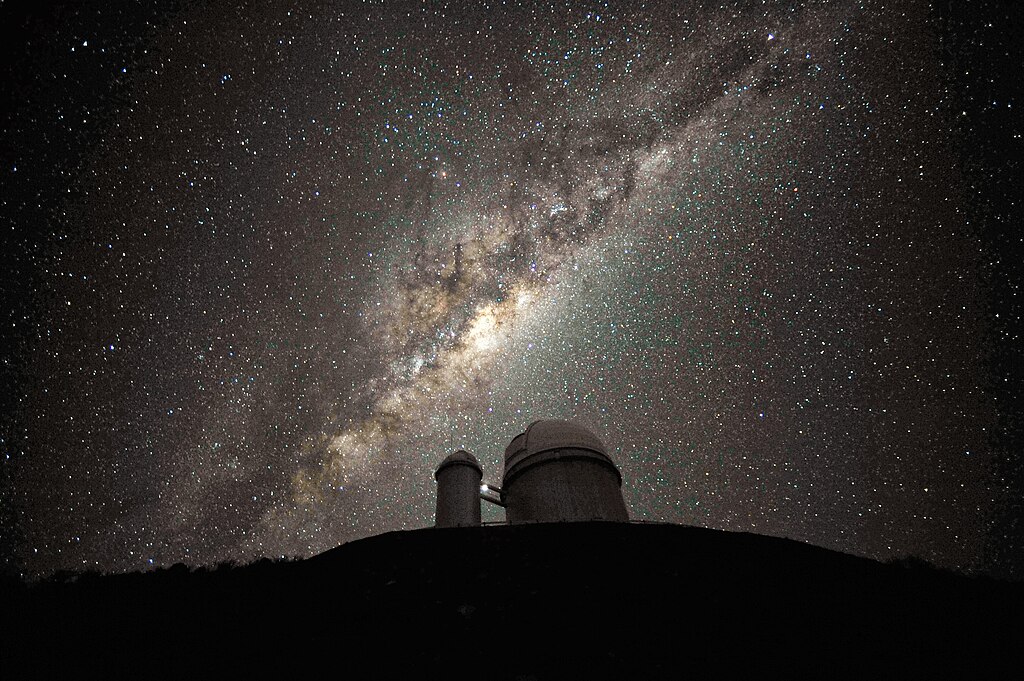
Understanding Machine Learning's Role in Astronomy
Scientists in South America and Italy have discovered significant limitations in a machine-learning algorithm that astronomers typically use for analyzing vast datasets of celestial objects.
Key Findings
- This algorithm successfully identifies many types of astronomical objects.
- However, its performance falters with hard-to-see galaxies.
- The findings suggest a pressing need for alternative analytical methods.
Conclusion
The study illustrates the powerful yet flawed capabilities of machine learning in the field of astronomy. As scientists continue to push the boundaries of data analysis, improving algorithms will be essential for better classification accuracy.
This article was prepared using information from open sources in accordance with the principles of Ethical Policy. The editorial team is not responsible for absolute accuracy, as it relies on data from the sources referenced.