Addressing Hallucinations in Generative AI: Solutions and Strategies
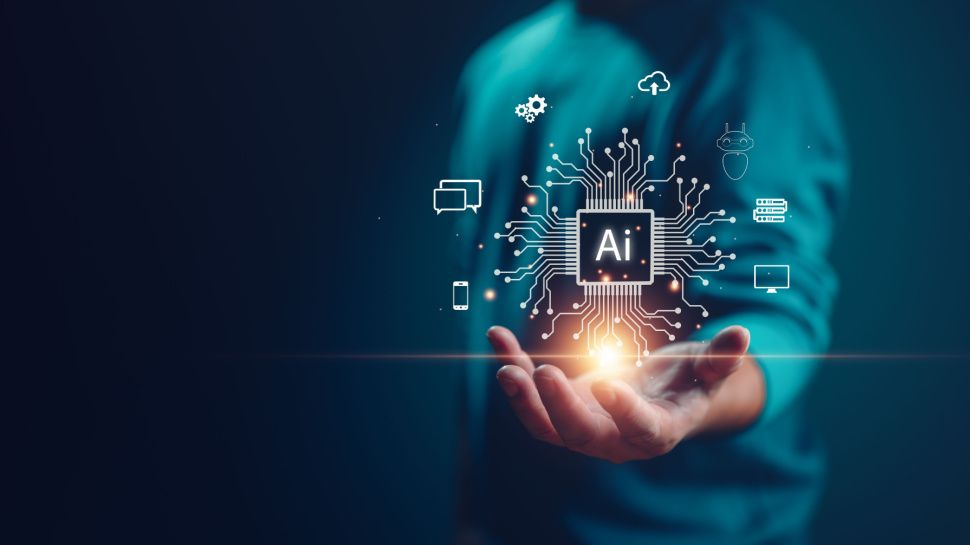
Understanding Hallucinations in Generative AI
Generative AI models intrinsically exhibit hallucinations, where the outputs generated may not align with real-world data. This phenomenon can lead to misleading results, potentially impacting decision-making processes.
Causes of Hallucinations
- Data Bias: Incomplete or unrepresentative training data can skew AI outputs.
- Model Architecture: Certain model designs may be more prone to generating inaccuracies.
Strategies for Mitigation
- Improving Data Quality: Ensuring diverse and high-quality datasets are used for model training.
- Algorithm Refinement: Enhancing the underlying algorithms to reduce error rates.
- Human Oversight: Implementing checks by human experts can validate AI outputs.
Conclusion
Addressing hallucinations in generative AI is essential for improving its reliability and effectiveness. By deploying robust strategies, developers can create more trustworthy AI applications.
This article was prepared using information from open sources in accordance with the principles of Ethical Policy. The editorial team is not responsible for absolute accuracy, as it relies on data from the sources referenced.