Utilizing Deep Learning for Accurate Infant Age Estimation from Skull X-ray Images
Thursday, 18 July 2024, 09:38
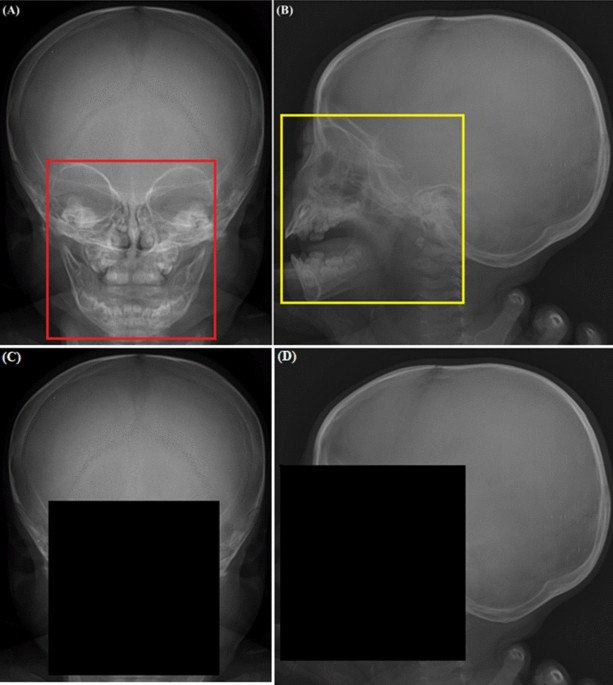
Utilizing Deep Learning Models for Infant Age Estimation
This study employed state-of-the-art convolutional neural networks, DenseNet-121 and EfficientNet-v2-M, to predict the postnatal age of infants using X-ray images of their skulls.
High Accuracy Rates Achieved
- Accurate Predictions: The models reached maximum corrected accuracy rates of 79.4% to 87.3% for different views, showcasing their effectiveness.
- Key Areas Identified: Critical areas on the skull X-ray images like sutures and bone density were pinpointed for age estimation.
Precision in Age Estimation
- Non-Invasive Methods: Deep learning offers precise age estimation without invasive procedures, aiding in clinical diagnostics.
- Developmental Assessment Tools: The study paves the way for improved tools for developmental assessments in infants.
This article was prepared using information from open sources in accordance with the principles of Ethical Policy. The editorial team is not responsible for absolute accuracy, as it relies on data from the sources referenced.