Role of Morphometric Characteristics in Predicting Children's 20-Meter Sprint Performance: A Machine Learning Study
Thursday, 18 July 2024, 06:53
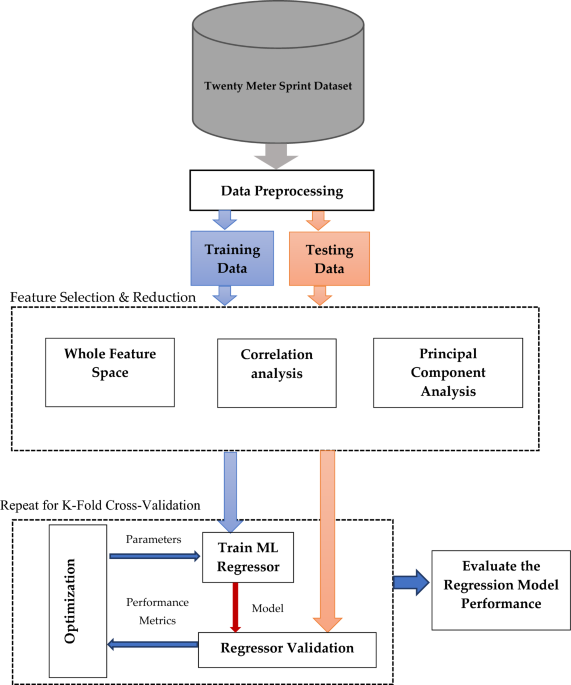
Study Overview
This study examines morphometric features' impact on children's 20-meter sprint performance using machine learning techniques.
Participants
- 130 males and 152 females aged 6-11 years were involved in the study.
Experiments Conducted
- Initial training on entire feature space.
- Feature selection based on correlation analysis.
- Application of Principal Component Analysis for dimensionality reduction.
Key Findings: Correlation-based feature selection yielded accurate predictions with minimal error (MSE 0.012).
This article was prepared using information from open sources in accordance with the principles of Ethical Policy. The editorial team is not responsible for absolute accuracy, as it relies on data from the sources referenced.