Innovative Methods Enhance Lung Cancer Classification
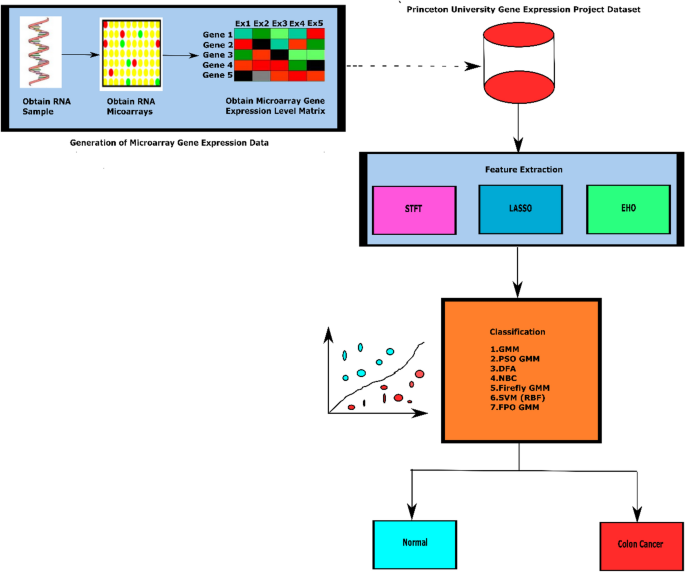
Challenge of Microarray Gene Expression Data
The microarray gene expression data presents a challenge due to the curse of dimensionality problem, causing issues with overfitting and reduced classification accuracy.
Significance of Feature Extraction Methods
STFT, LASSO, and EHO are utilized to extract meaningful information and reduce the dimensionality of the microarray gene expression data.
Impact on Lung Cancer Classification
By employing innovative methods like EHO feature extraction and FPO-GMM classifier, the research achieves high accuracy, F1 score, MCC, and Kappa values.
This article was prepared using information from open sources in accordance with the principles of Ethical Policy. The editorial team is not responsible for absolute accuracy, as it relies on data from the sources referenced.